Sql2Odi – best practices for auto-generating your ETL content
I blogged a while ago about our Sql2Odi tool that converts SELECT
(and WITH
as well) statements into Oracle ODI Mappings. (Blog posts 1, 2 and 3.)
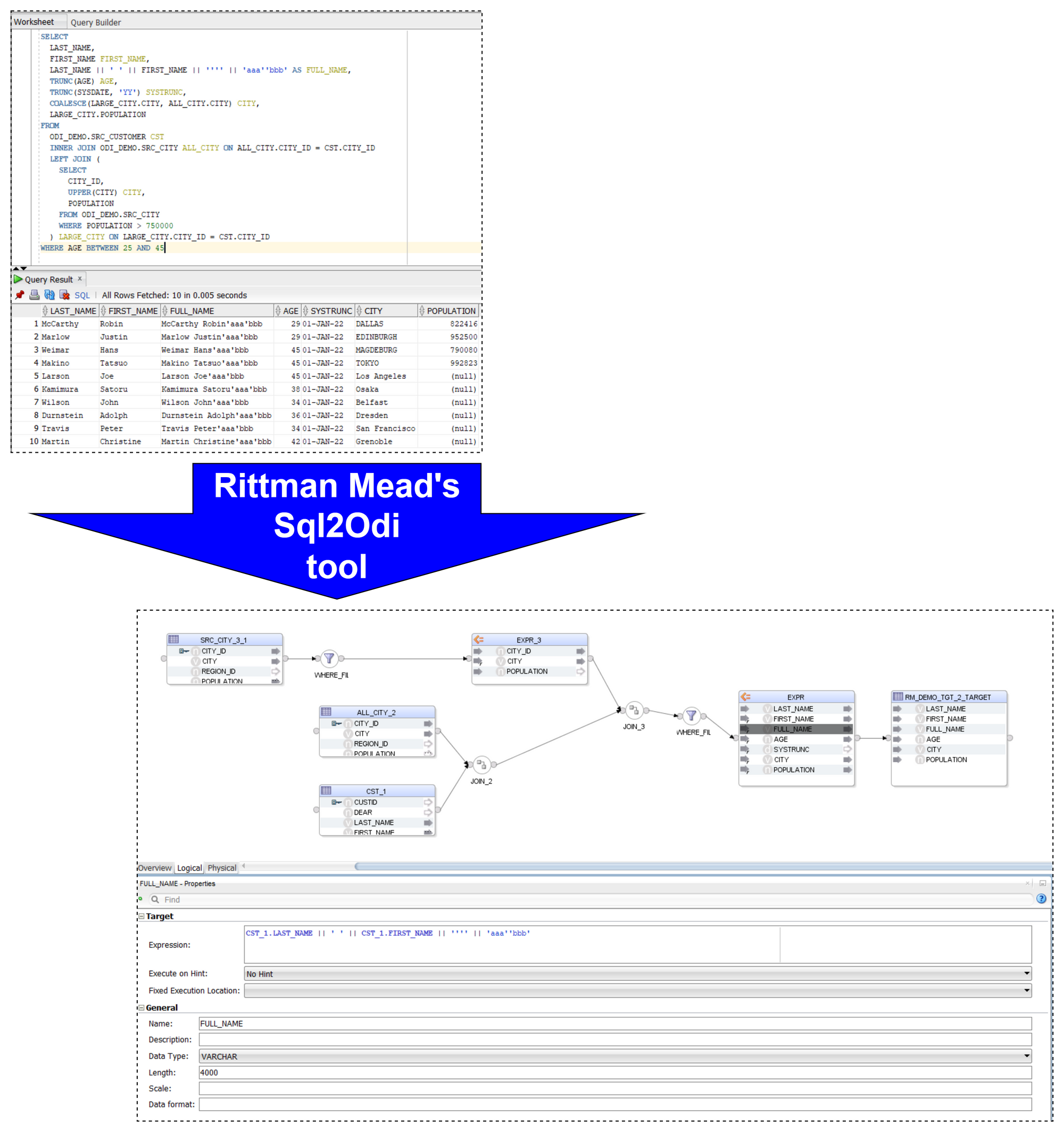
Now that the tool has been around for close to 2 years, let us reflect on lessons learnt and best practices adopted.
Let me start with a quick reminder of how the Sql2Odi tool works:
- You start by defining the feed-in metadata: the SELECT statement that the new Mapping will be based upon, name of the target table, names of Knowledge Modules and their attributes, flags like Validate Mapping and Generate Mapping Scenario flag, etc.
- Then run the Sql2Odi Parser that parses SELECT and WITH statements in your metadata table.
- Then run the Sql2Odi Mapping Generator that creates ODI Mappings based on the successfully parsed statements.
- Assess the outcome by reviewing the newly generated Mappings in ODI Studio.
Defining Metadata
Each SELECT statement that we want to turn into an ODI Mapping needs its own line in the SQL_TO_ODI_MAPPING
metadata table. (It currently has 35 columns and is likely to grow in the near future as the tool's capabilities are expanded.) It takes time to fill in all metadata required for Mapping generation. If there are many Mappings to be generated and they can be split into groups according to purpose and design pattern, for each group you should create its own custom metadata table with its unique distinguishing features. Once all the custom metadata tables are filled in, based on them you can generate records in the SQL_TO_ODI_MAPPING
table.
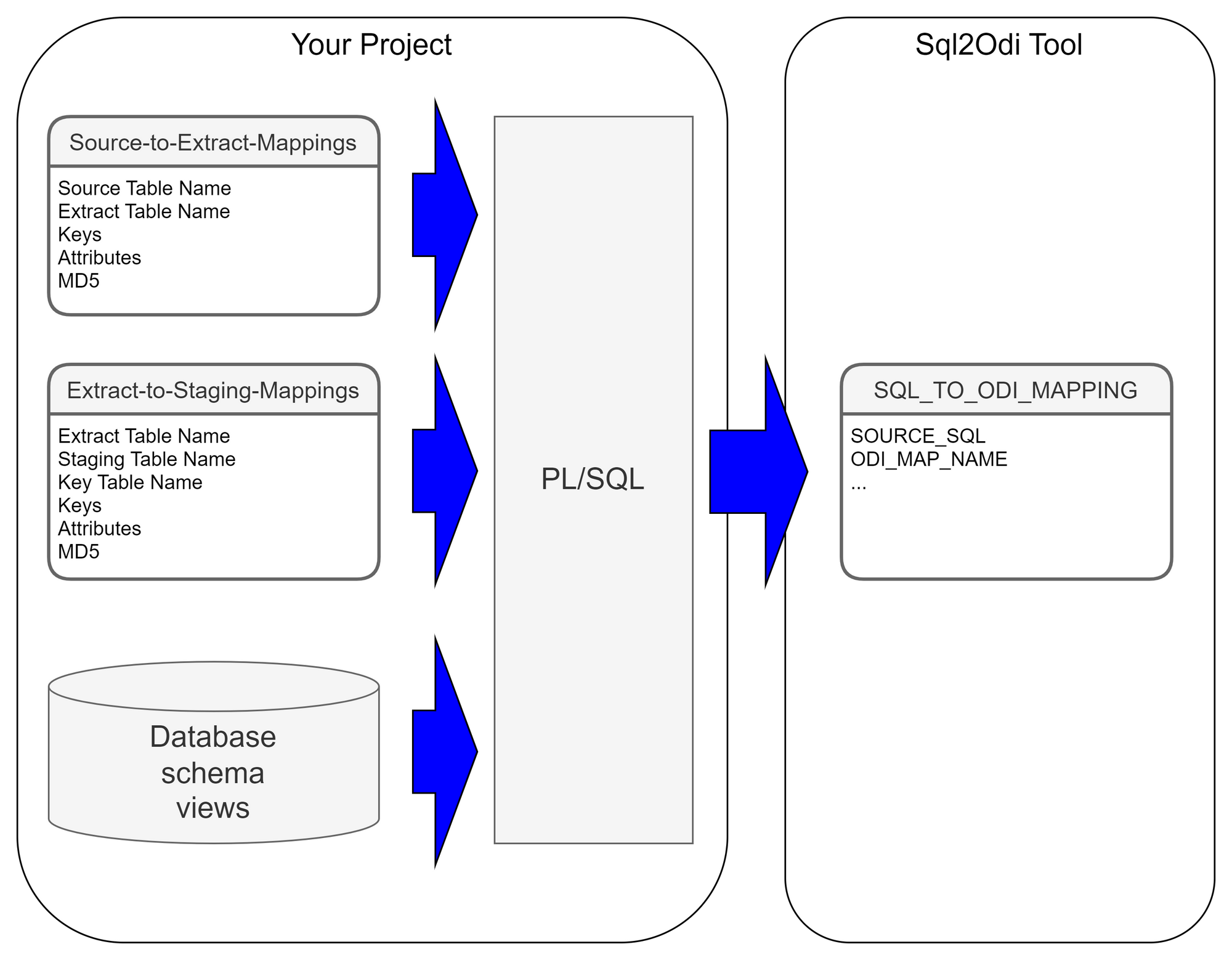
Executing Parser
Sql2Odi Parser parses SELECT and WITH statements and in case of a successful parse converts it into metadata that in turn is used by the ODI Content Generator to generate Mappings in ODI.
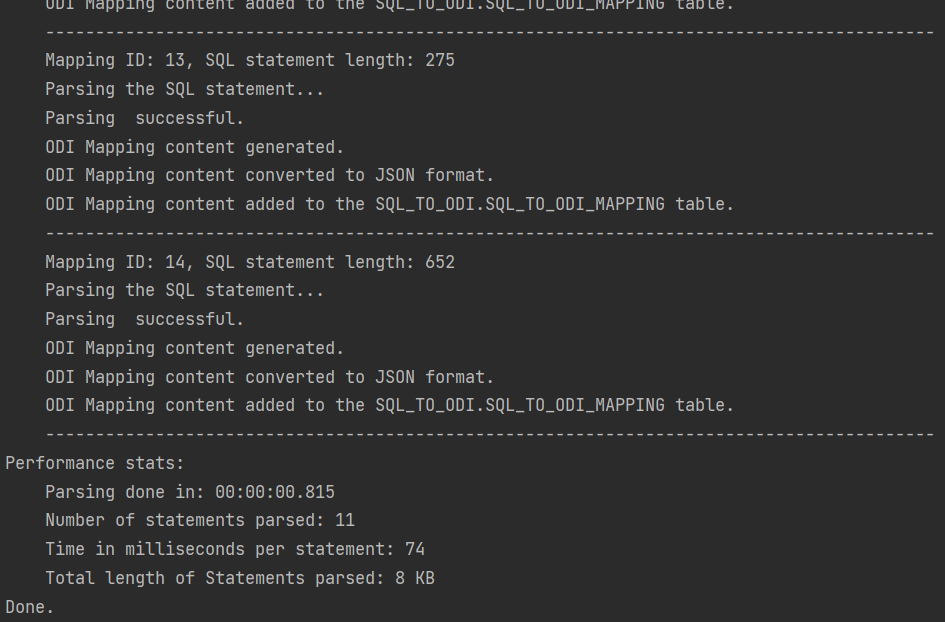
Any reserved word that is part of the SELECT statement, needs to be defined in the Parser so that it can understand it - that is something that can only be done by us. (The Parser does not have visibility of the database schema, does not validate a SELECT statement against actual schema content.) Any custom-built PL/SQL functions will hence not be understood. Standard Oracle RDBMS functions are supported but occasionally we are still finding some obscure ones that we have missed. Our Parser still has a range of limitations:
- All reserved words, including Oracle function names, must be given in UPPERCASE. (This is usually not a problem, because SQL Developer allows to convert any SELECT statement to uppercase.)
- No support for nested queries (where a SELECT substatement is used instead of a column reference or expression).
- No support for the
EXISTS()
expression. - No support for hierarchical queries, i.e.
CONNECT BY
queries. - No support for
PIVOT
queries. - No support for the old Oracle
(+)
notation. (You can still join tables in theWHERE
clause if you so choose butOUTER JOIN
should be used where in the old days we used(+)
. - Does not parse comments unless those are query hints.
Like most parsers, ours is not good at telling you what and where exactly the problem is. For example, if in your SELECT statement you are referencing a custom-built function, the Parser will most likely interpret it as a column name and will become upset when you try to pass values to it.
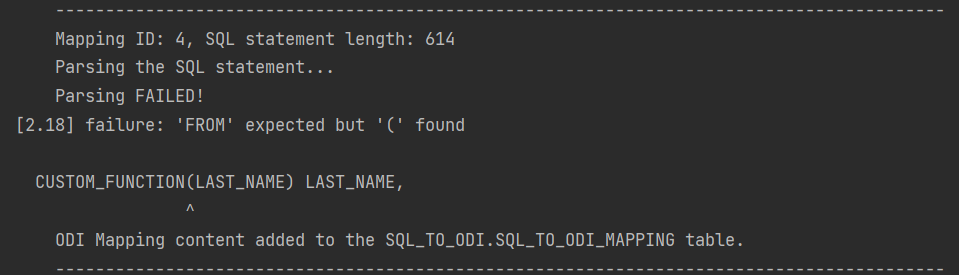
It will say it expects something else instead of the opening bracket (
whereas the problem is actually the function itself. Therefore it is a good idea to test and review all SELECT statements before passing them to the Sql2Odi tool.
Executing ODI Content Generator
ODI Content Generator is a Python script that you run from ODI Studio. It will generate new Mappings in the dev repository that you are currently connected to.
As was noted above, the Parser does not know anything about the database schema you are referencing. So if you have not tested your SELECT statement in SQL Developer, there might be typos in your table or column names - all those typos will be parsed successfully and those issues will come to light when you try to generate Mappings in ODI: if a schema name is incorrect in the SELECT, the ODI Model will not be found, if a table name is incorrect, the ODI Datastore will not be found, if a column name is wrong, attribute mappings will fail to be created.
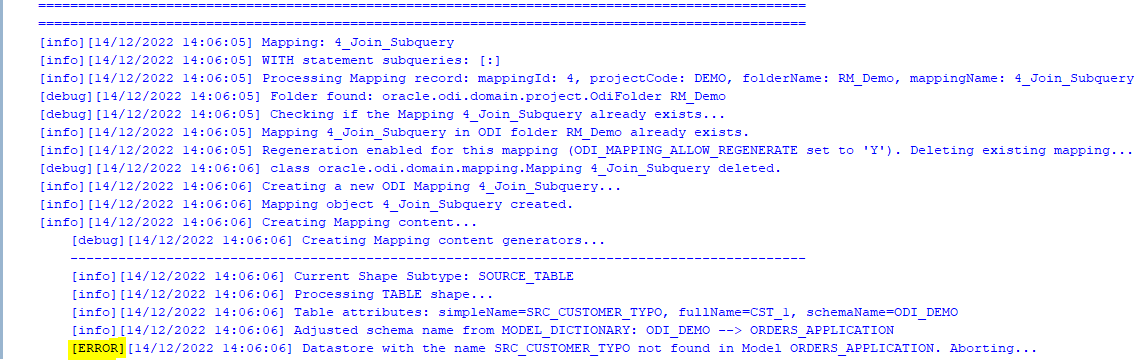
However, the good news is that the ODI Content Generator is much, much better at telling you what, where and why went wrong - it generates a rich log output and shows descriptive error messages.
Because semantic errors can only be spotted upon trying to generate Mappings, many cycles of edit SELECTS -> parse -> generate ODI content
may be necessary.
When Mapping generation is done, always check the output log for errors and warnings!
Using Sql2Odi in a Project
The ODI Content Generator by default will delete and recreate Mappings, thus allowing for many generation cycles. However, this can be overridden at the level of individual Mapping - there is a flag in the SQL_TO_ODI_MAPPING
metadata table: ODI_MAP_ALLOW_REGENERATE_FLAG
. If set to N
, Mappings that are already in the Repository, will not be touched.
In a project where the Sql2Odi tool or any other auto-content generator is used, there should be a well understood milestone for generated content, beyond which it passes into the domain of developers for further manual adjustment and can no longer be re-generated. When that milestone is passed, will depend on how far the Mapping can be developed by automated means. Typically it will take many cycles of auto-generation to get Mappings in the desired shape. How many cycles we will run, will depend on the review feedback after each generation but also on the performance of the auto-generator tool.
Performance
One of the benefits of using auto-generators is - making a small adjustment to all objects of same type is easy, for example, adjust MD5 calculation to include Source system ID for all Extract Mappings, of which there could be thousands. But will it take 5 minutes or 5 hours to regenerate the lot? A five minute job can be done almost any time whereas a 5 hour one looks like a nightly job. To discuss the performance of the Sql2Odi tool, we need to look at the Parser and the ODI Content Generator separately.
The Parser is a pure Java application with few outside dependencies. On my test system, it takes tens of milliseconds to parse a single SELECT statement. That suggests that parsing a thousand SELECT statements should be done within a minute. Also, parsing does not interfere with anything or anyone.
Parser performance stats:
Parsing done in: 00:00:01.675
Number of statements parsed: 42
Time in milliseconds per statement: 39
Total length of Statements parsed: 61 KB
The ODI Content Generator however is a different story. ODI content generation based on the parsing result written in the metadata table is done by a bunch of Groovy scripts, which query and update the ODI Repository repeatedly in the course of creating a single ODI Mapping, and they are subject to object locks in ODI Repository. Content generation is much slower than Parsing and its performance will depend heavily on the speed of your dev environment, how complex the Mappings are, do you set up Physical Design, validate and create Scenarios as part of creation, etc. On my test system it takes a couple of seconds for a Mapping to be fully generated, validated, Scenario generated.
[info][12/12/2022 19:10:26] -= Performance Stats =-
[info][12/12/2022 19:10:26] Of 38 enabled and successfully parsed Mappings in the Metadata table, 18 Mappings were successfully generated.
[info][12/12/2022 19:10:26] Total duration is 45.5 seconds, which is 2.527 seconds per successfully generated Mapping.
The Future is Automation
Let us sum it up. The way your accelerated, automated development process will work will depend on the constraints like the complexity of your SELECT statements, the amount of manual intervention needed after your Mappings are generated and the performance of the automation system. You have designed your data analysis system and concluded that large parts of your data warehouse schema and the ETL (or ELT) can be built automatically. The Sql2Odi tool enables you to do that.
Why Data Governance Is More About Enabling Good Than Stopping Bad
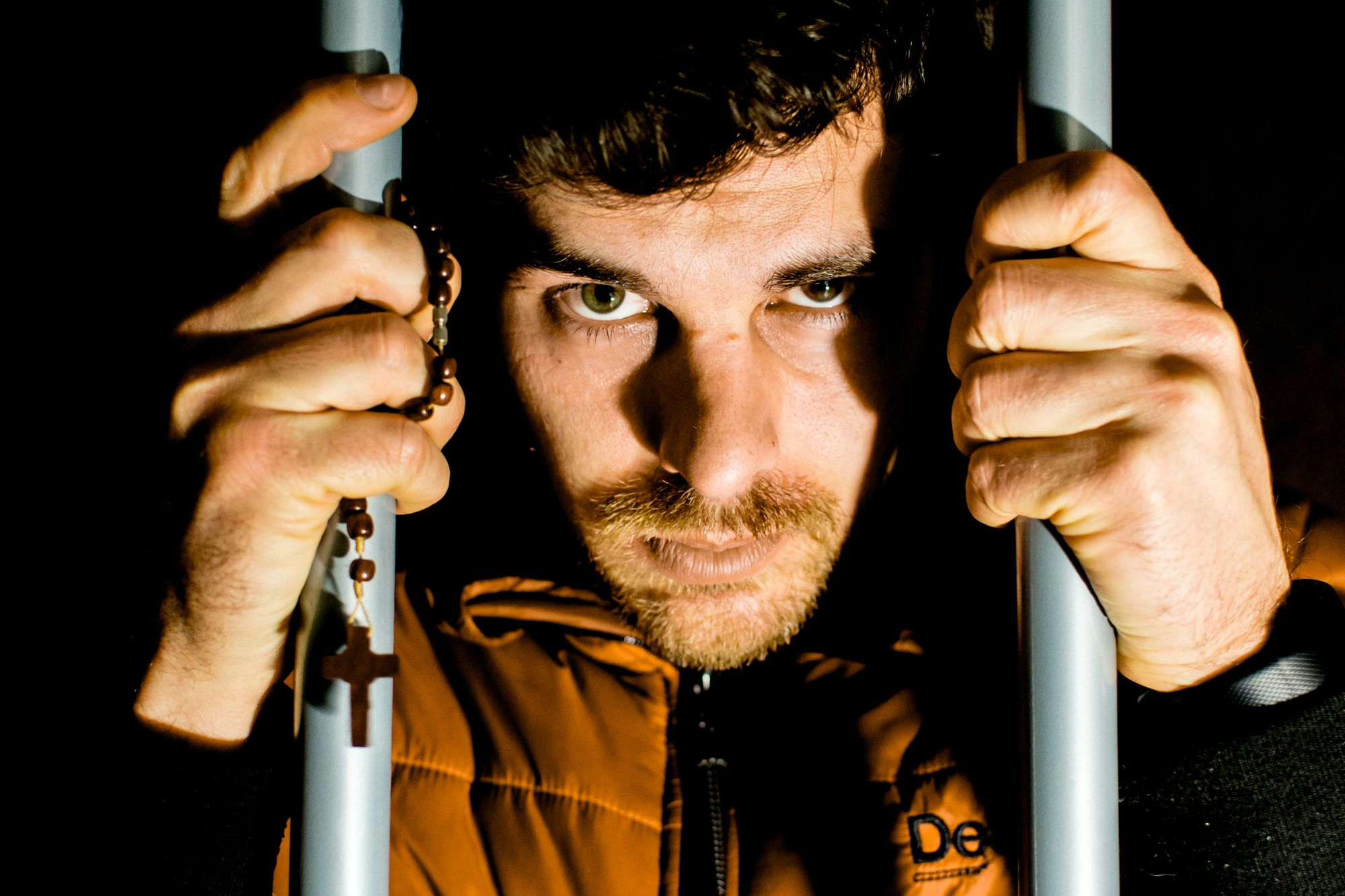
It seems like every day businesses are bombarded with reminders of the value of data. Data, we are told, leads us to make smarter decisions that deliver better outcomes. No arguments there.
But we are constantly reminded of the dangers of data too; of the consequences of losing it, accidentally or through malicious actors. Attacks on Stuxnet, Equifax and the NHS are just some of the cautionary tales we share around. The cost of each attack is an eye-watering $4.5m, estimates IBM in its Cost of Data Breaches Report 2022. Add in the fallout from reputational damage, and the level of cybersecurity investment needed to protect against increasingly sophisticated threats, and a cost-benefit analysis of using data may conclude that it’s just not worth it.
It is common practice for businesses to address this risk with a data governance framework. As such, data governance is often presented as preventive; the art of stopping bad things happening. That positioning continues to be its downfall. When a business frames data governance in terms of risk mitigation and regulatory obligations, it becomes an ancillary function. It can quickly be superseded by activities that have a more immediate impact on the bottom line, be it revenue generating or cost cutting measures. When trading conditions are tough, this inclination to deprioritise data governance in favour of perceived core business endeavours is even stronger.
Instead, data governance should be seen as enabling good; that with the right controls and processes, you improve the quality of data you have; get it to the people who can gain most value from it; and so drive better outcomes with it.
This thesis supports the trend for self-service data discovery and BI. Businesses increasingly understand that data by itself is fairly useless. Data needs context, and so it becomes more powerful when in the hands of those making business decisions. This decentralised approach requires a level of organisation around who can access and share different data. That should be a fundamental aspect of a data governance framework—not because it’s important to stop data getting into the wrong hands, but to make sure the right hands get the data they need. Equally, a data catalogue and data management tools should be built with the end-user in mind, making it easy for them to find, understand and interrogate the data they need. In contrast, many businesses organise their data to demonstrate regulatory compliance.
Data also needs to be credible. In other words, do users believe the information and insights they are being given? So data governance must provide assurances on data provenance and establish control processes and tests to ensure data validity. Again, the emphasis here is on a framework that enables rather than restricts. When an executive trusts their data, they can make clearer, bolder decisions with it.
Culture is also key to an effective data governance framework. In the self-service, decentralised world of BI, data is no longer the sole remit of one team. Data and analytical literacy must be embedded into everyone’s role and every workflow. So data governance should not be seen simply as a framework for information security. It is also a set of behaviours and processes that are designed to foster collaboration at all levels and instil confidence in users as much as to keep them and the company safe. Data governance should describe how this culture permeates the entire business, from leadership through to individual lines of business, and right down to how every employee handles and uses data and information.
Transparency should be elevated to a core value. Mass data participation won’t be achieved if users are worried that they’ll do something wrong. But when vulnerabilities are valued and mistakes seen as a learning moment, users have no need to be paralysed with worry. Data becomes a playground of discovery. A data governance framework must bake this culture in from the start.
The traditional view of data governance is as a block to information and innovation. Executives want to be set free, to explore data and the secrets it surfaces. But a data governance framework that priorities regulation and security puts in place policies and protocols designed to restrict independence. Rather, effective data governance should be the reason you can say “yes” to data requests.
Often the problem is that data governance is considered too late. Insert data governance over existing priorities, policies and processes, and it is seen as being intrusive. But start with a data governance framework, and design everything else around that, and it becomes ambient, quietly enabling, never compromising.
3 elements of an effective data governance
Lead from the top
Executives responsible for data governance must set the right tone. Excite employees about what an effective data governance can do for them, not what it will stop them doing.
Governance by design
A governance framework that truly liberates data must permeate the entire business, including systems, processes and culture. Architect governance with this scope in mind from the start.
Opportunity-based approach
A data governance framework should define the outcomes it wants to achieve. Resist the temptation to take a risk-based approach. Instead, describe the opportunities that effective data management can deliver.
Oracle APEX – Debugging Tip #1
If I would need this feature I would probably invest a lot of time trying to find out an answer. But funny enough I found out by mistake and as it might be useful to someone else I have just decide to publish this short blog post.
Application Process on Global Page
When working with Report queries Oracle APEX creates an URL that you can use in your buttons or branches to call the report itself. That URL uses the global page 0 with an special REQUEST syntax as showed below.

I used that URL in a branch that fires when the "PRINT_REPORT" button is pressed:
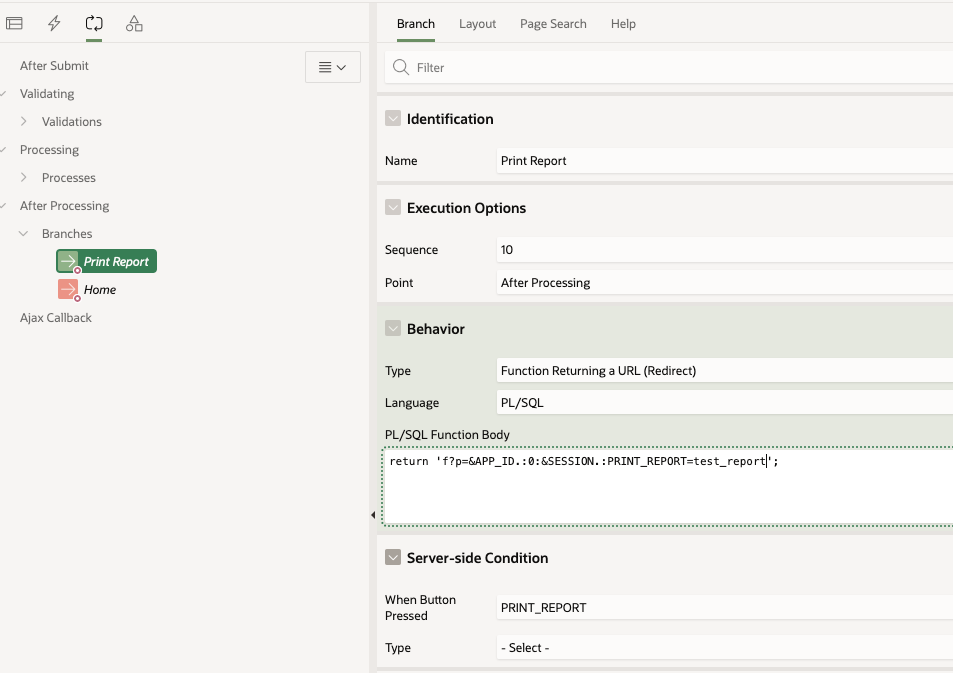
While trying to debug this process you might run into the following problem. If you enable debugging from the Developer toolbar like this:
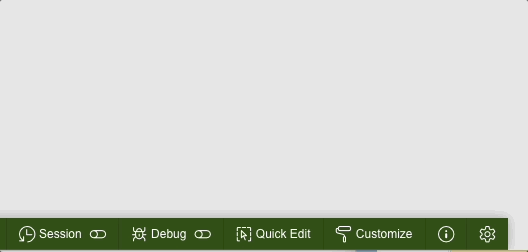
or from the URL:

the page 0 process won't be debug. The output of the debug trace from the "View debug" option will be limited to the call of the button on page 1.
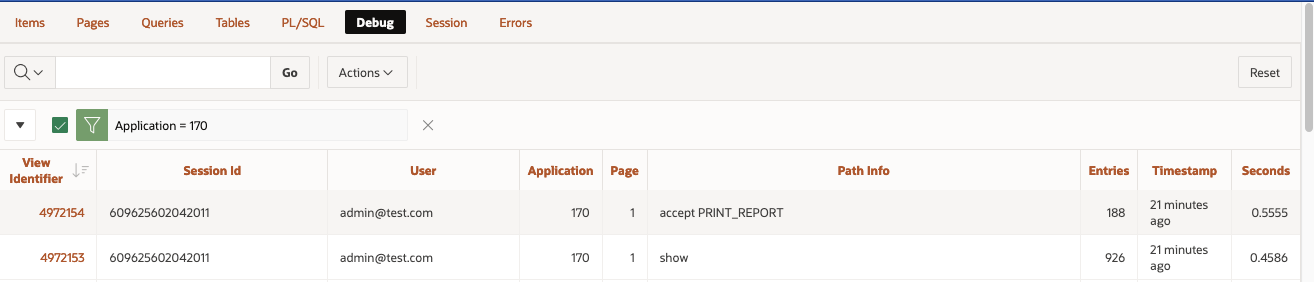
Same goes for the Session Activity view:

On then contrary, if you enable debug from Monitor Activity > Active Sessions > Session Details
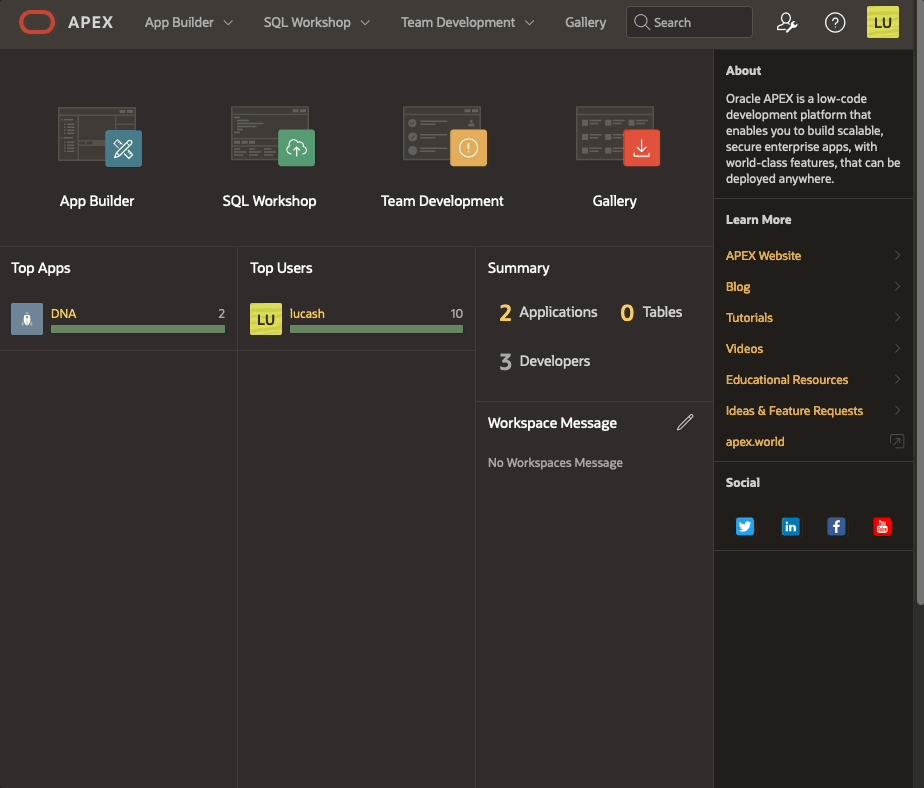
Debugging happens as well in page 0 processes. The "View debug" show the debug line:
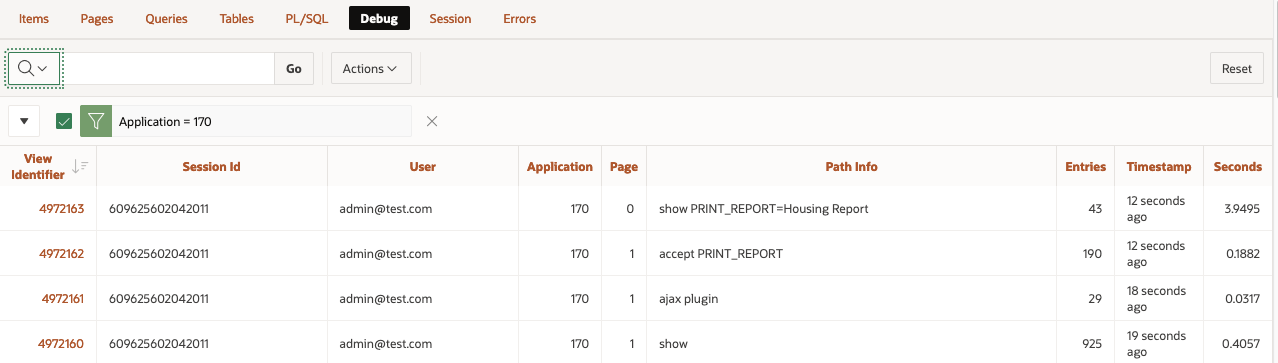
Same information is displayed in the Session details:

Oracle APEX bug or feature?
Not sure if that is a bug or a feature and don't think is relevant at this stage. The important bit here (and applies for every aspect of the development cycle) is that we get to know different ways of reaching the same end, different methods to try, to keep us going forward :)
We’ve added Search!
Oracle APEX – Social Login
I looked into Social Sign-in as an option for Oracle APEX a few years ago. This was pre APEX 18.1 and, at this time, it was not simple to configure (in fact it would have taken a considerable amount of code to implement)
Fortunately, since 18.1 APEX offers natively functionality to integrate with Single (Social) Sign on providers and makes the whole process much easier.
This blog will describe the process of getting it up and running and why this might make life easier for both the developer and as an end-user.
Why rely on a 3rd party identity provider (IdP)?
Using a 3rd party to manage and authenticate the users that will be accessing your APEX application offers several potential advantages.
Firstly, delegating such a crucial task as security to an expert in the field in authentication is an inherently sensible idea. It eliminates the need to support (and possibly code for) a password management system inside the APEX application itself. This relieves an APEX developer of time spent managing users and worrying about the innate security risks that go hand in hand with storing this type of data. Not to mention trying to implement Two-Factor-Authentication (2FA)!
Secondly, from a user's perspective it should provide a better experience, especially if the IdP is chosen carefully based on the application's use. For example, if the application is to reside within an enterprise environment where users are already using Microsoft Azure to authenticate into various services (such as email) then, using the Azure IdP APIs, users could login into APEX with the same username / password. If the APEX application is deployed in a more publicly accessible space on the web, then using a generic IdP like google / facebook will allow you to capture user details more simply, without exposing users to the tedious experience of having to type in (and remember) their details for yet another website to enable them register or pay for something.
Allowing users to login to many systems using a single 3rd party system is sometimes know as federated authentication or single sign on (SSO) and the choice now includes many providers
- Microsoft
- Oracle IDCS, Ping, Okta etc

How do they work?
The protocols IdPs use to authenticate users into client applications (such as APEX) have their roots in Oauth2, which is a standard developed by the web community in 2012 to help websites share users' resources securely. A typical example of a requested resource is when a website (the client) you are registering on wants to access the list of contacts you have in your gmail account (the resource holder) so it can email your friends, in an attempt to get them to register too. Oauth2 allows an authorisation flow where the website can redirect you to a google server (the gmail provider) which will subsequently ask you to authorise this request and, with your consent, then provide an access token back to the original client website, which would be then used to query your contact data securely.
With Oauth2 websites could start sharing all sorts of data with each other, including, commonly, simple user profile data itself (eg name, email address, phone numbers). However, it is important to recognise that Oauth2 is an authorisation rather than an authentication protocol. In 2014 the Oauth2 specification was extended to include OpenId, which specifically deals with the authentication of a user. It is these standards that IdPs use to federate users.
The flow in more detail
The following diagram / points explain the data flow in more detail. In this example we will use a hypothetical set up a client app using Facebook as its IdP. Note that before this can occur the client app will have needed to register itself with Facebook and obtain a client id and client secret which it will need in some of the authentication steps
- User attempts to log onto the client. The authentication scheme redirects the user to Facebook authentication end point (with its client id)
- User Authenticates onto Facebook (if not logged in already). User prompted to confirm that he trusts the client app (this step is removed the next time the user logs in)
- Facebook redirects back to the application with an authorisation code
- The client application uses the authorisation code (with its client id and secret) to get and identity token about the user (with various meta data) and that is accepted by the client as a valid authentication
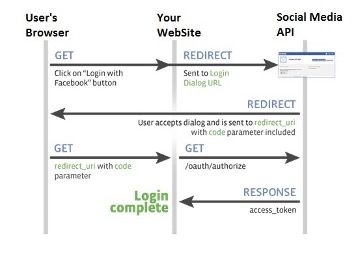
Setting up an example in APEX!
OK, so let’s get an example up and running. For simplicity, I shall run through doing this on the Universal Theme application. We will change access from Public to Social Sign in (OAuth 2.0), create a couple of tables to hold who and when they logged into the application, and then add a report page to the application to detail user access.
This assumes you have the following:
- An Oracle APEX Cloud account and have a workspace and the Universal Theme application installed (the latest UT application can be downloaded from here).
- You have created a Google Account which will require a credit card. You may have already used this to create your Free Tier ATP Instance where you have APEX installed and like this scenario, we will need it for Google. Unless you launch your app to a very large community and start using other Google API features it will be free too. Google will send you alerts if and when you approach the end of the free quota, which resets each month. As an example, Google Maps Matrix API allows 28k calls a month for free.
- You have some familiarity with APEX and are not a complete beginner. If you are just starting, I would recommend you use one of the many resources now available online to get started.
Oracle have a lot of information and here is a good starting point.
Overview of what we will build
- Open a developer account with Google & setup the Developer Account
- Register an application into this google account and generate a client id and secret
- Register the client id and secret in an APEX workspace
- Create an APEX app and set up a Goole based social sign in Authentication scheme
- Create an APEX Authorisation scheme with a number of steps following your demo with done additional explanations on the way were helpful. Maybe just install UT and change that to require Google Auth
- Extract the user’s name [maybe add gender, locale and picture] from the Google OAuth 2.0 call and store in app items. (Having the image where the APP_USER is located would be really cool)
- Build a few simple tables that hold the user details such as their internal ID, when they last logged in etc
- Discuss roles in the app to secure functions for different user types. Create an Admin page and some reporting on the access from the users. Implement this initially with an Authorisation Scheme such as SQL statement of 1=1
- Introduce IDCS and roles and demonstrate setting this up with the Administration role
(do we need a standard role too for a user who comes in with the Google creds but is not an Admin?) - Moving back to the UT application, we will modify the authentication and authorisation to provide this function
The “How To” Build Steps
Finally! Here is the hands-on fun bit!
Open a developer account with Google & setup the Developer Account
Once you have a Google account (you already may have one if you use Gmail) you will need to navigate to the Google developer console.
If you have not done this already enter your details such as your address and mobile phone number. Use a mobile number as it makes life easier with confirmation text messages.
You will need to agree to Google’s terms and conditions and select whether or not to receive email updates. In terms of billing, it is probably a good idea to receive email updates but, in any case, you can opt out of this later if you want to.
You should now get to the following screen:
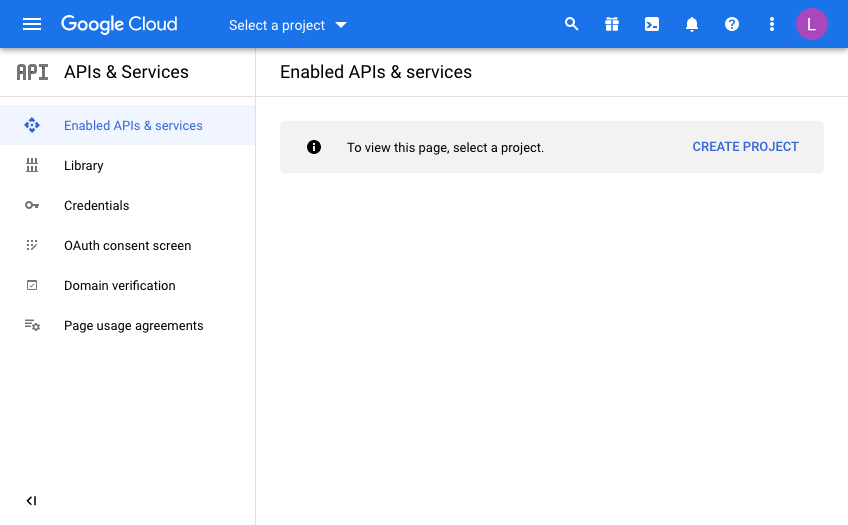
Every Google API call you want to make will be defined from a “Developer Project”. In doing this, Google makes it nice and easy to control and report on where your API useage is, which is important when have more than one project on the go.
This is useful for demos or switching off access to a system independently of others if you need to so that you have the ability to switch off some usage while leaving others unaffected.
Click “Create Project” and give your project a name. This will be pre-filled and you will need to either be creative or just use the ID as a suffix for example to make it unique.
I’m afraid that now means you can’t use “My Project 50628” as I have below!
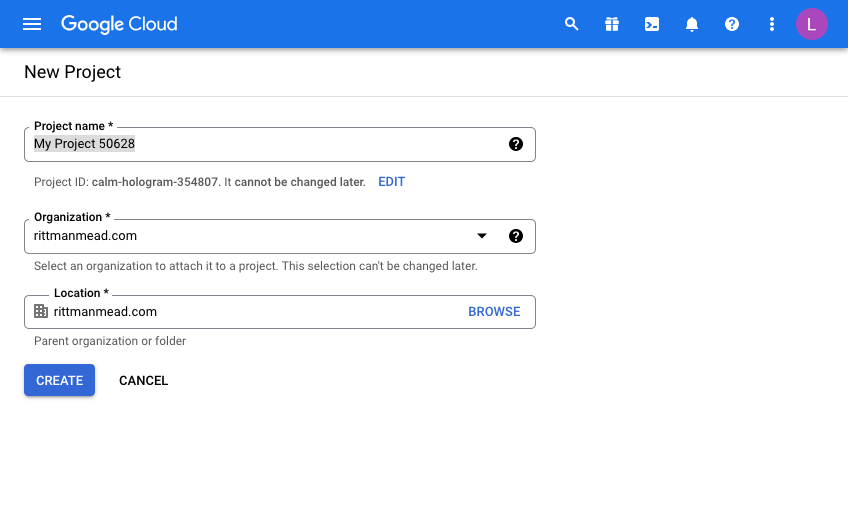
Once you have a project, you will have to configure the consent screen.
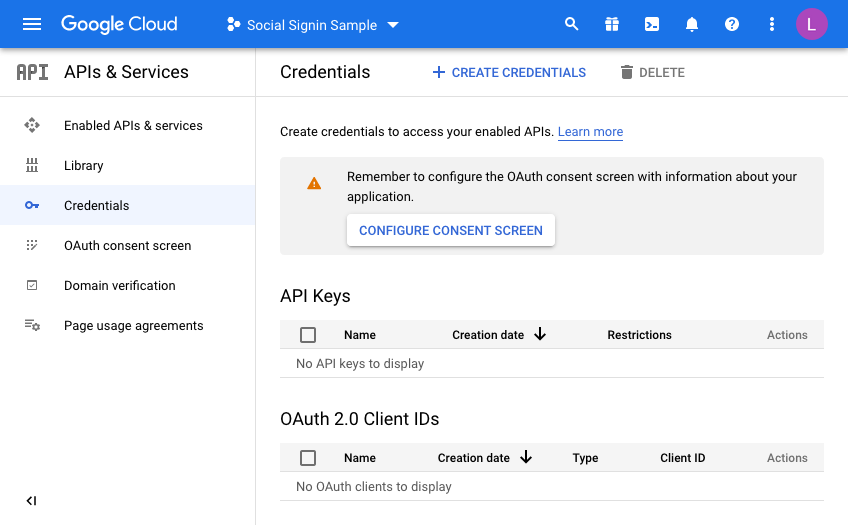
We’re going to make this available to all users with a Google account so select “External”
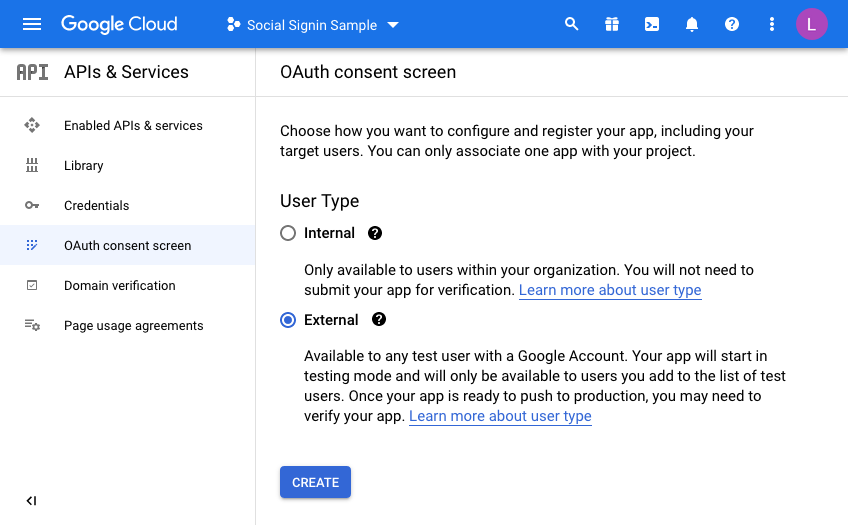
Enter details to match your setup:
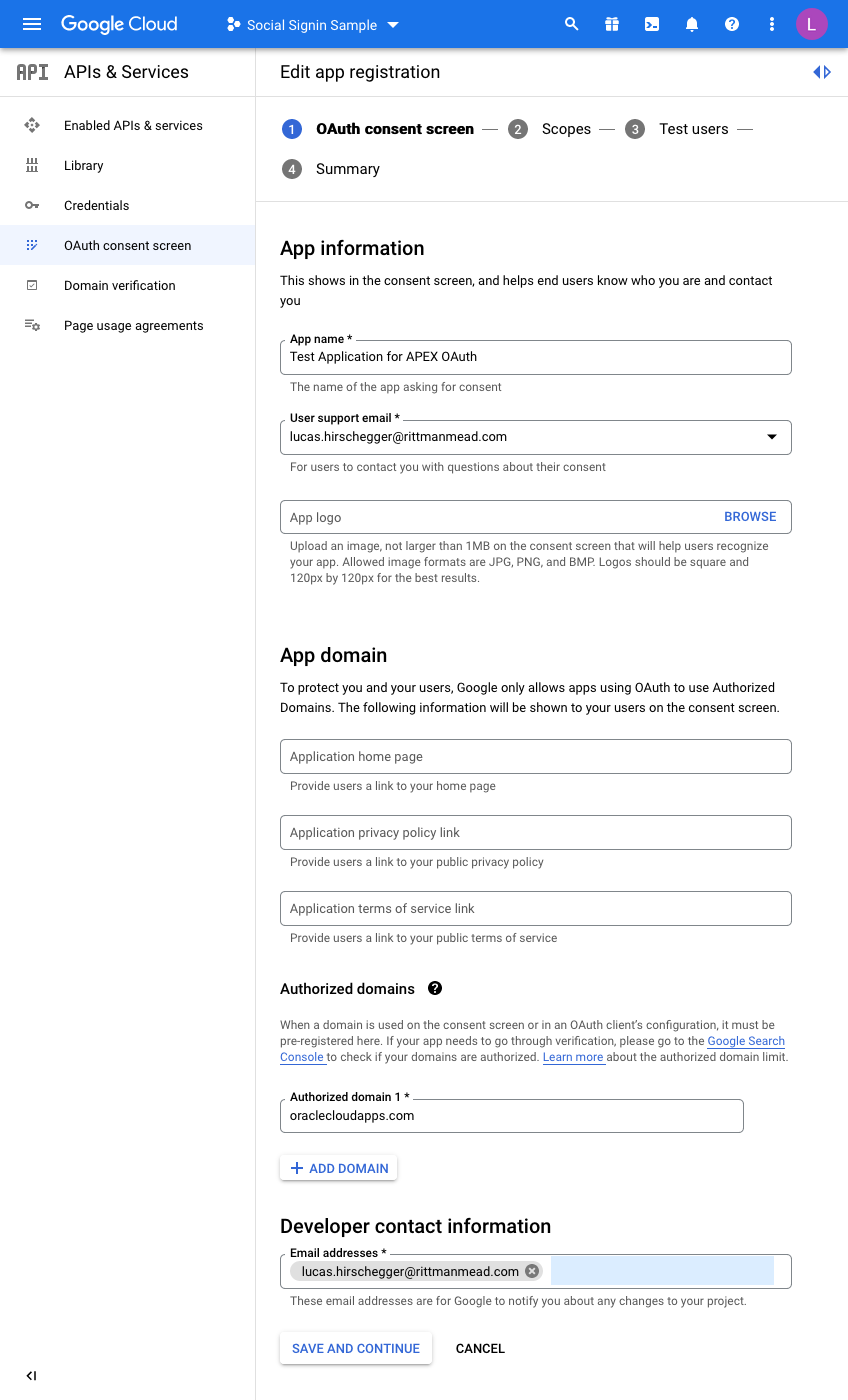
I have just entered the mandatory fields here. For the domain, just enter the base domain for the site so that my ATP Always Free Tier APEX home page is as follows and the base is bold:
https://xxxxxxxx.oraclecloudapps.com/ords/r/development/ut/home
The domain is oraclecloudapps.com in this example.
A Scopes configuration page is then loaded:
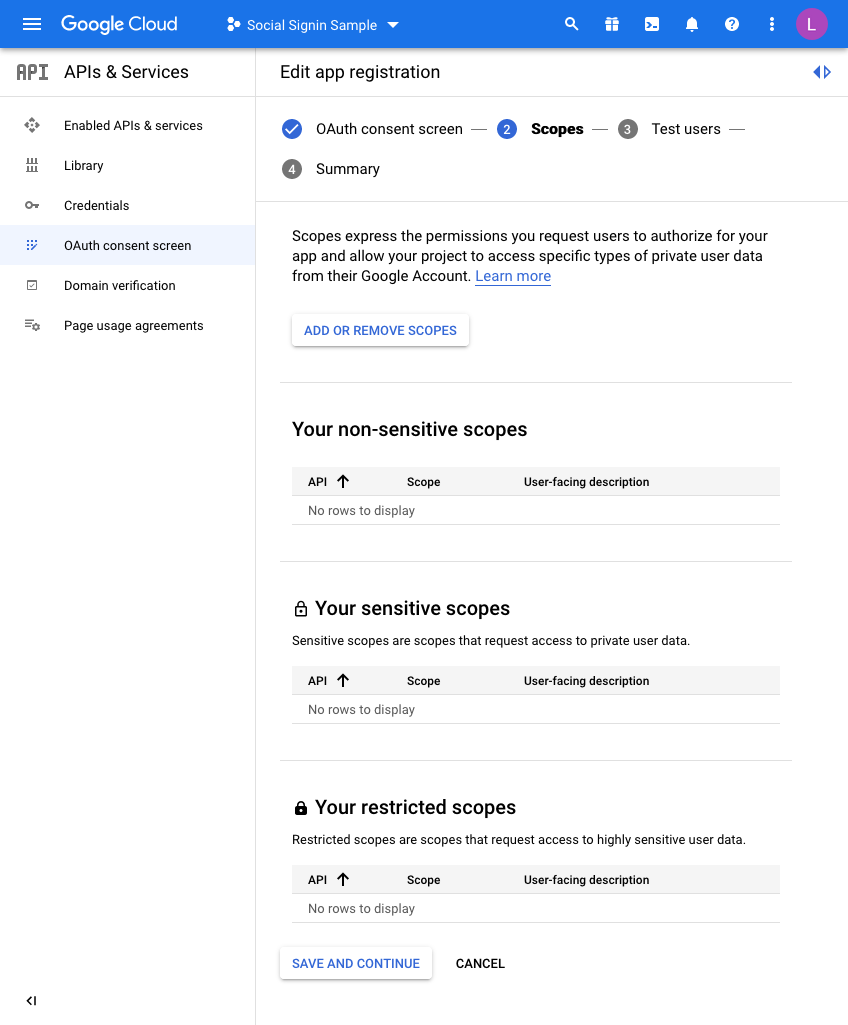
For this example, we are not going to set any scopes here so click
SAVE AND CONTINUE once again.
Finally, we are taken to a “Test users” page. Here you may choose to initially set your access to be limited to yourself & and limited set of users.
Unless you want to do this, click the SAVE AND CONTINUE button again. This project is only for the UT application so we do not mind sharing this without any test users defined as access is normally unrestricted (Public access in the APEX authentication scheme).
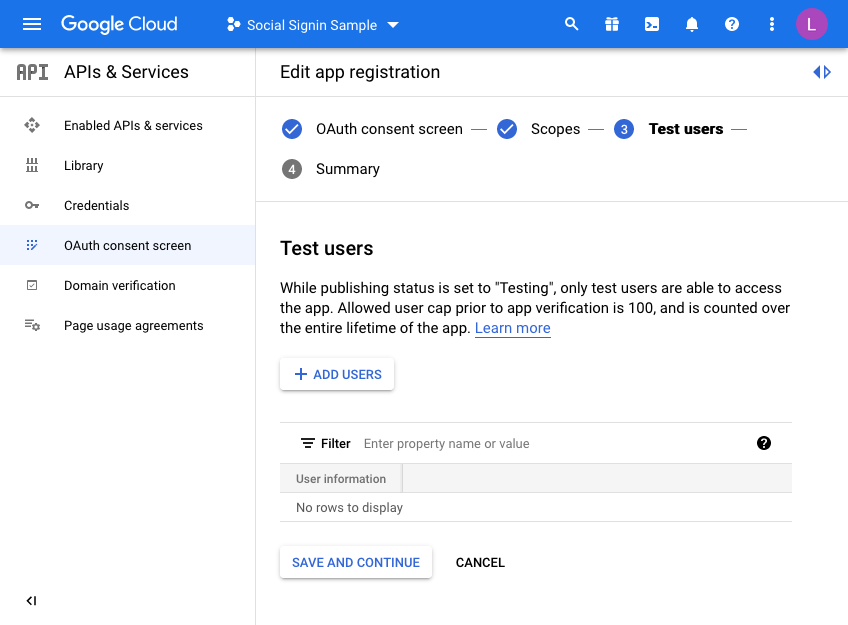
The last step is just the summary of the OAuth consent screen where you can double check the entries you have configured so far:
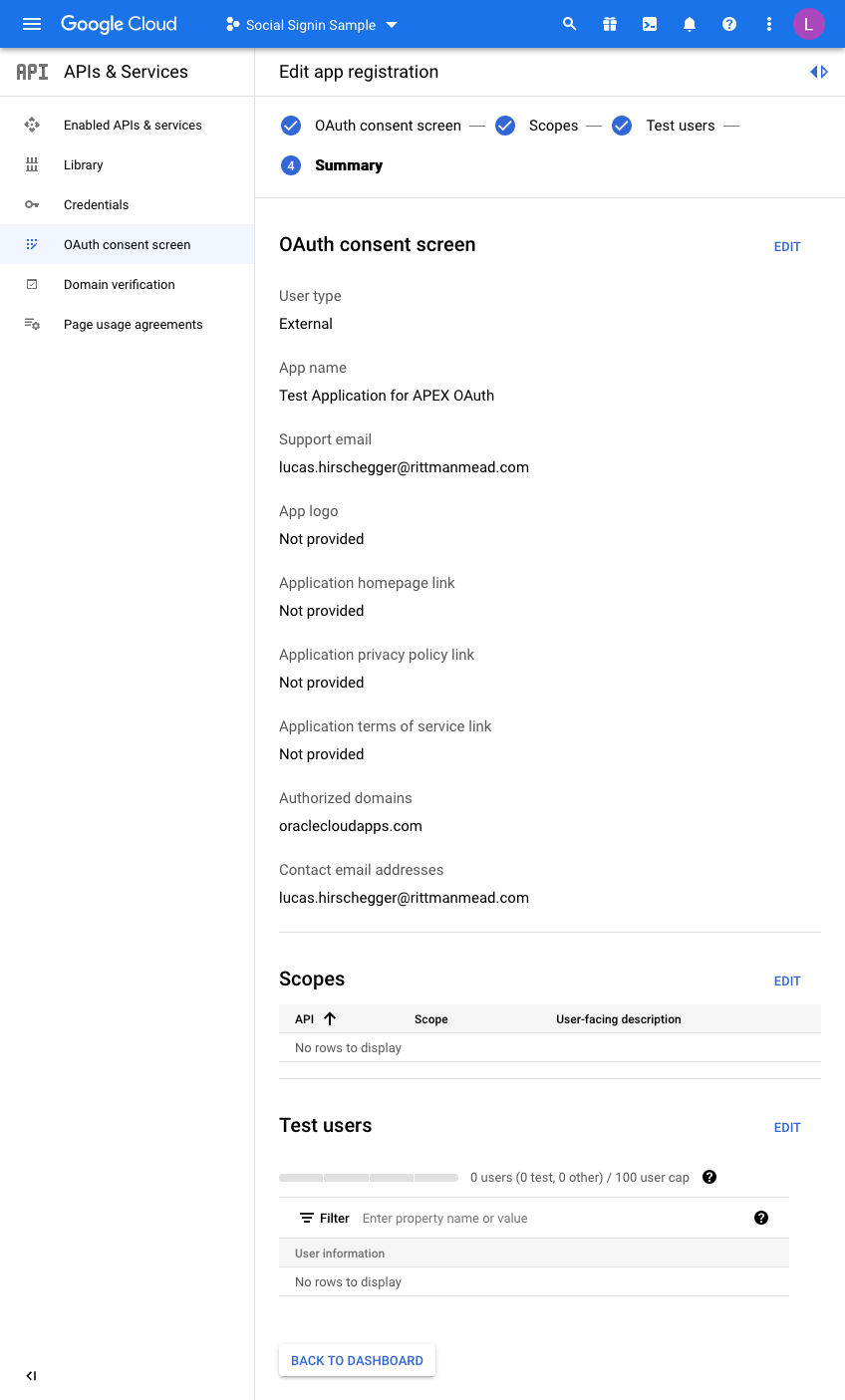
So now we are almost there on the Google side of things. We just need to generate credentials. Click on Credentials and then click CREATE CREDENTIALS, choosing OAuth Client ID in the dropdown menu:
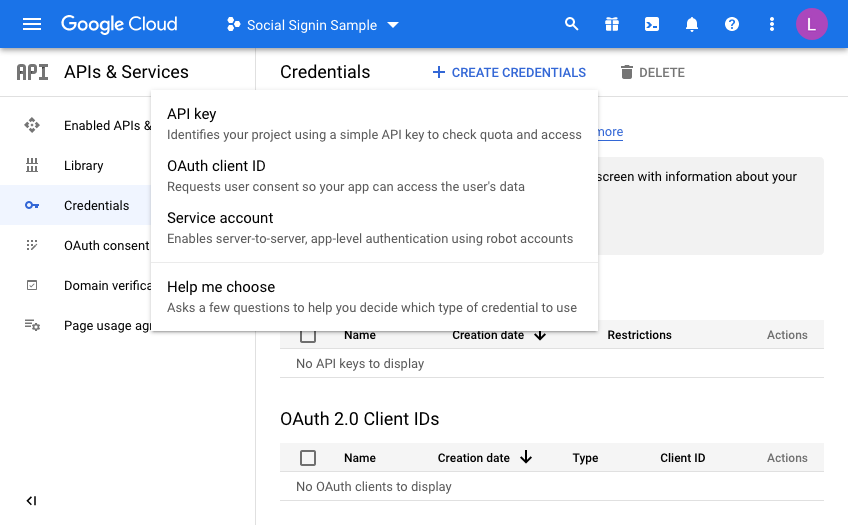
On the next screen we choose a “Web Application” and then fill in the name you wish to assign this set of credentials:
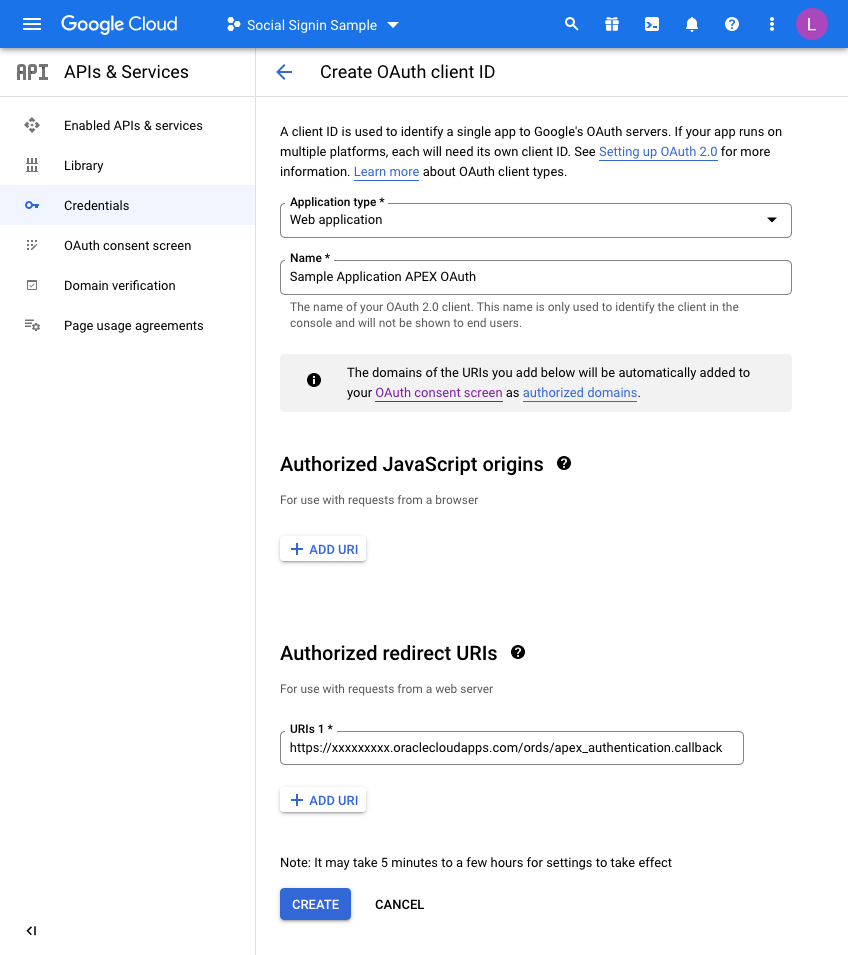
Before you click CREATE, add a redirect URL by pressing the ADD URI button.
All APEX redirects use the same redirect function which is just the part of the URL of your application and then an additional suffix.
Take the URL of any page in your UT Application (you can just run this if you are unsure) and then copy the URL up to the ords section and then add the extra string of:
apex_uthentication.callback
Specifically, in my example this is:
https://xxxxxxxx.oraclecloudapps.com/ords/apex_authentication.callback
Once this is defined, press CREATE and that competes the Google Integration setup.
A dialog will be presented with the following information. This is what we will now set up in APEX for the Universal Theme Application:
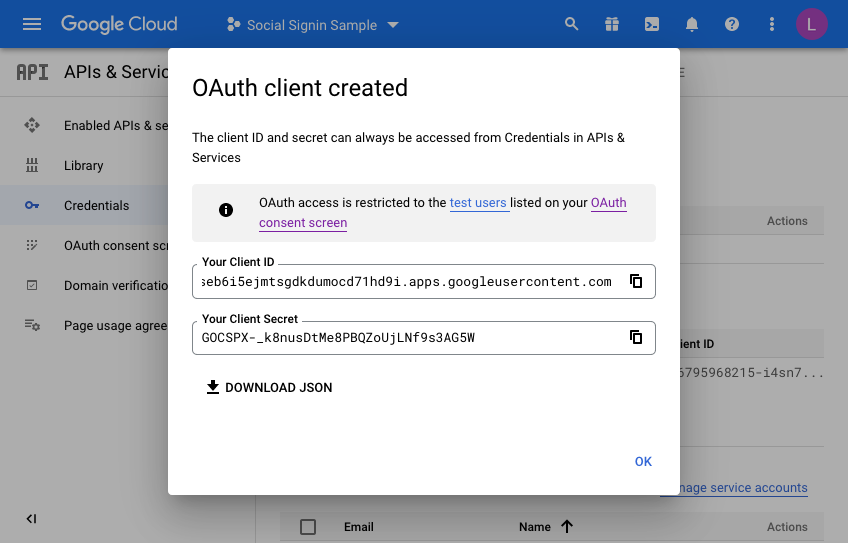
Make a note of the client ID and the Client Secret. We will need these when creating the APEX web credentials in the next section. Press OK a final time and you may now review what you have set up on the Google side of things.
Setting up the APEX environment - Web Credentials
The first step here is to define your web credentials in the APEX Workspace itself. Click on the "App Builder>Workspace Utilities > All Workspace Utilities" menu option:
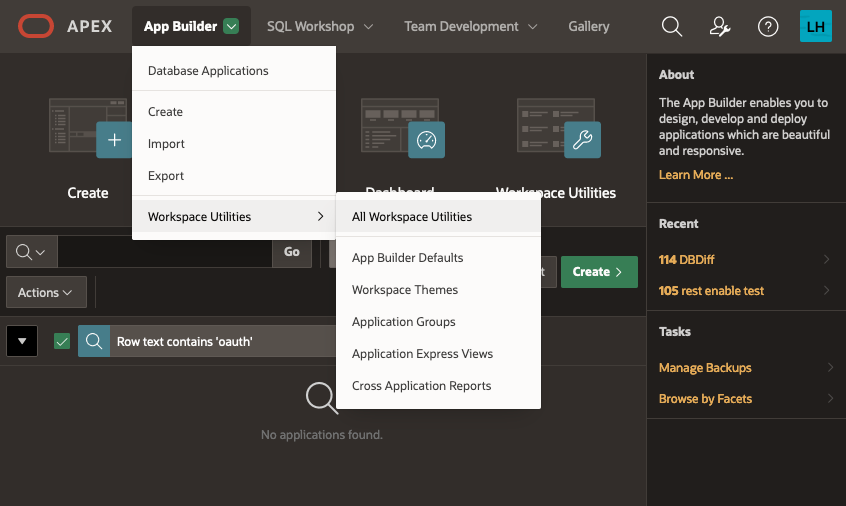
Next, choose “Web Credentials”:
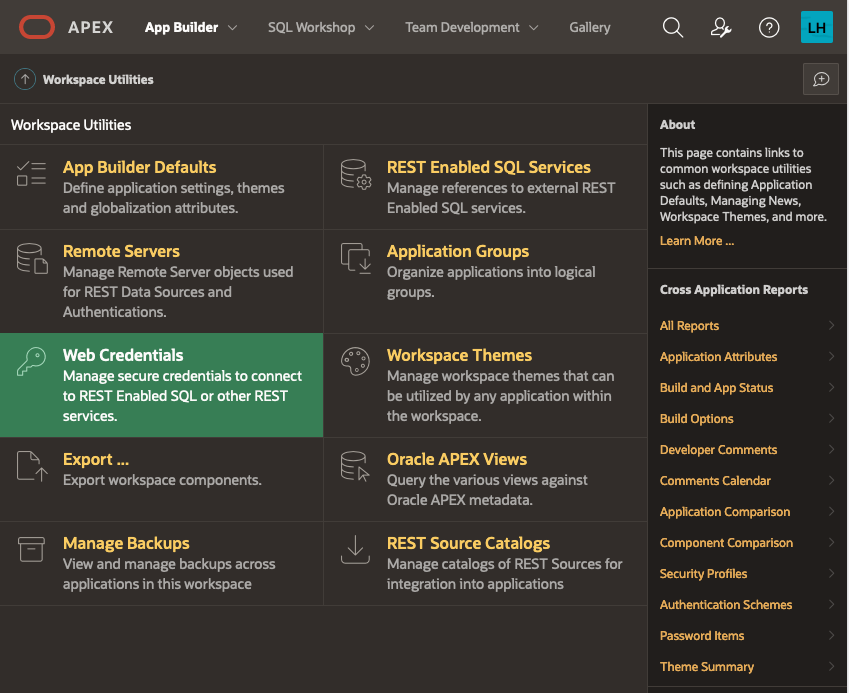
The list of credentials is shown, click on CREATE
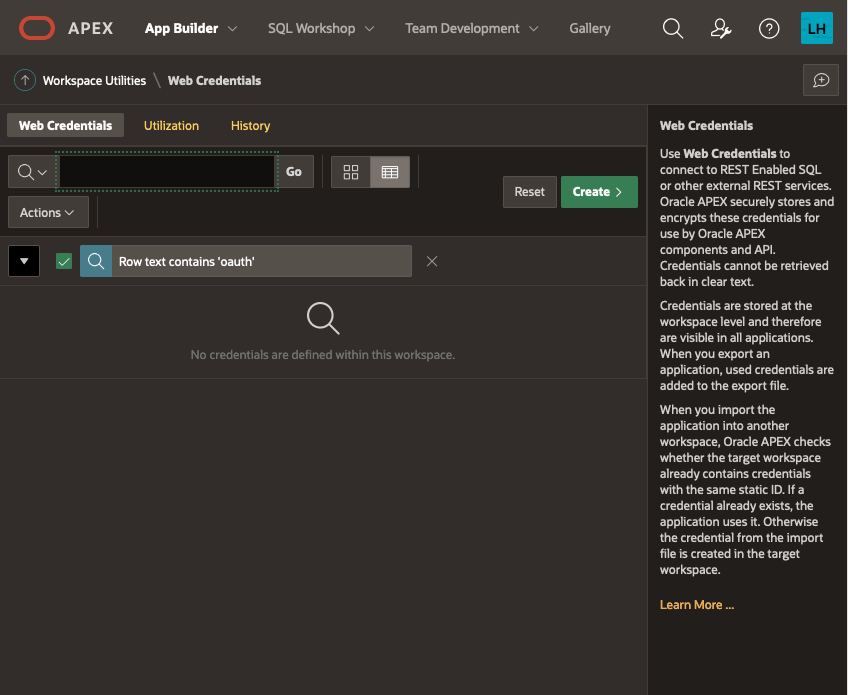
Give your Web Credentials a name and enter the Client ID and Client Secret from above repeating the secret in the verify field before saving these details:
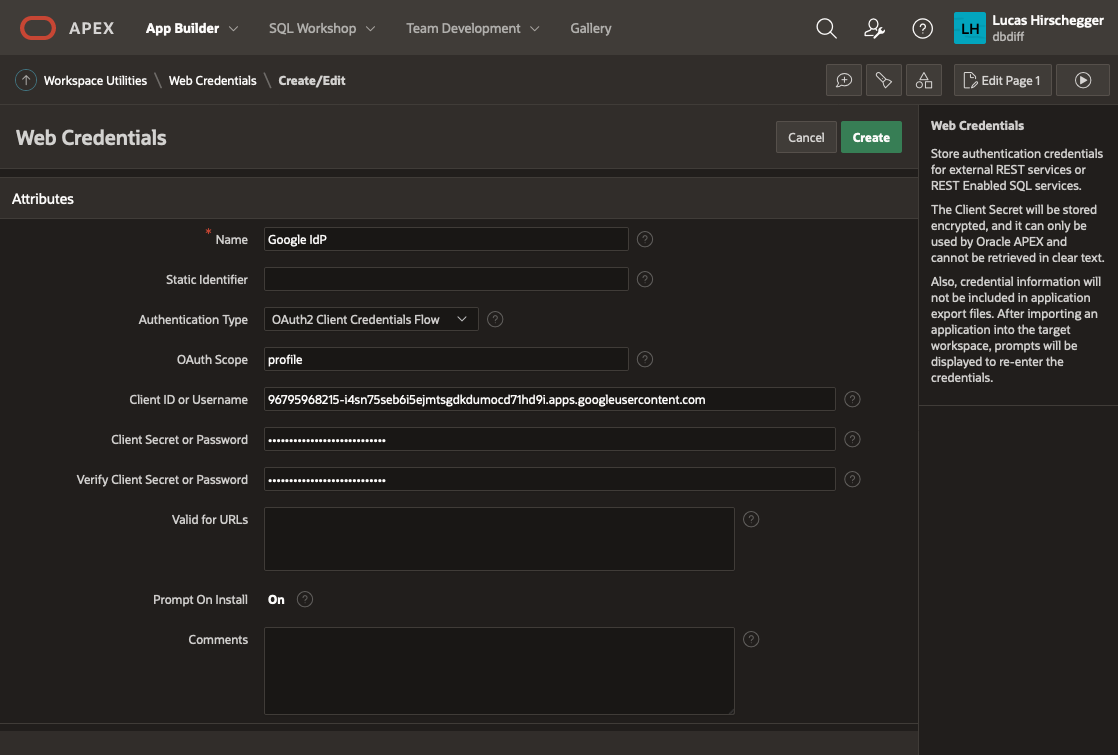
This is now available to any application within your APEX workspace. Now we shall use this for our UT application. I am assuming you have installed the UT application or maybe you are setting this up for an application you have developed but you will need an APEX application at this point. If you already have the UT application and use it for reference, you may want to copy it so that you keep one version that does not require authentication via Google OAuth2.
Go to application builder and navigate to the UT application you have installed.
Open it in application builder and then select
Shared Components>Authentication Schemes and then click CREATE.
Select “Based on a pre-configured scheme from the gallery” from the radio group and press NEXT
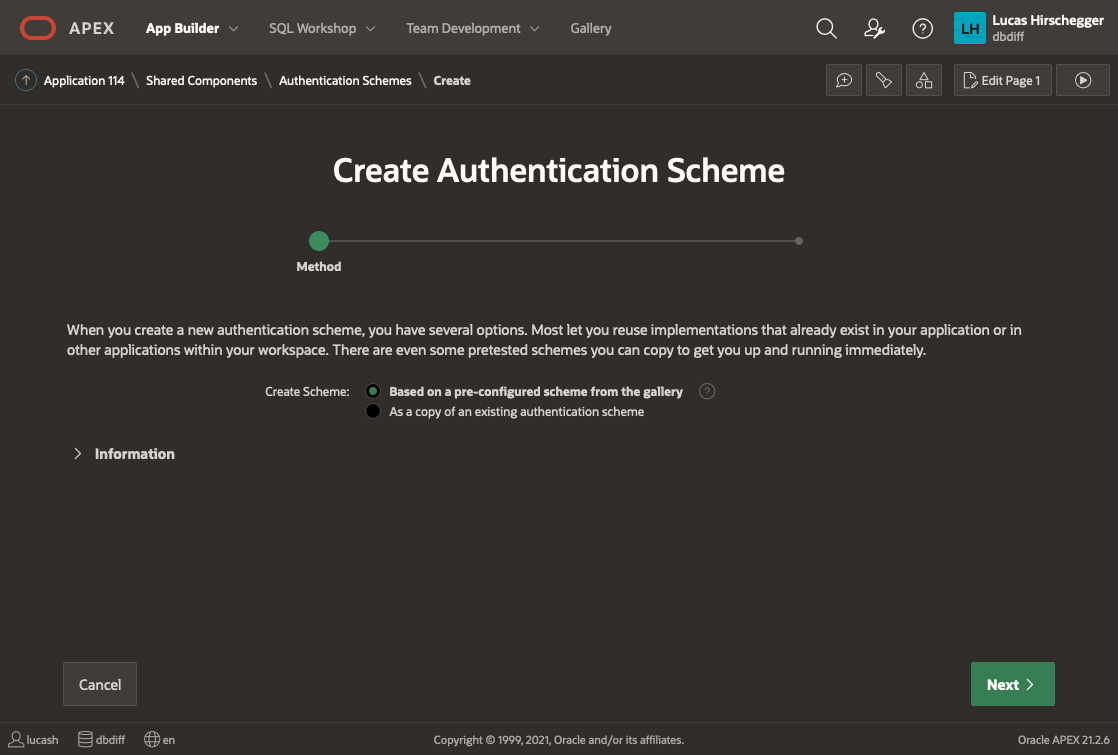
Now provide a name of your choice and select “Social Sign-in”:
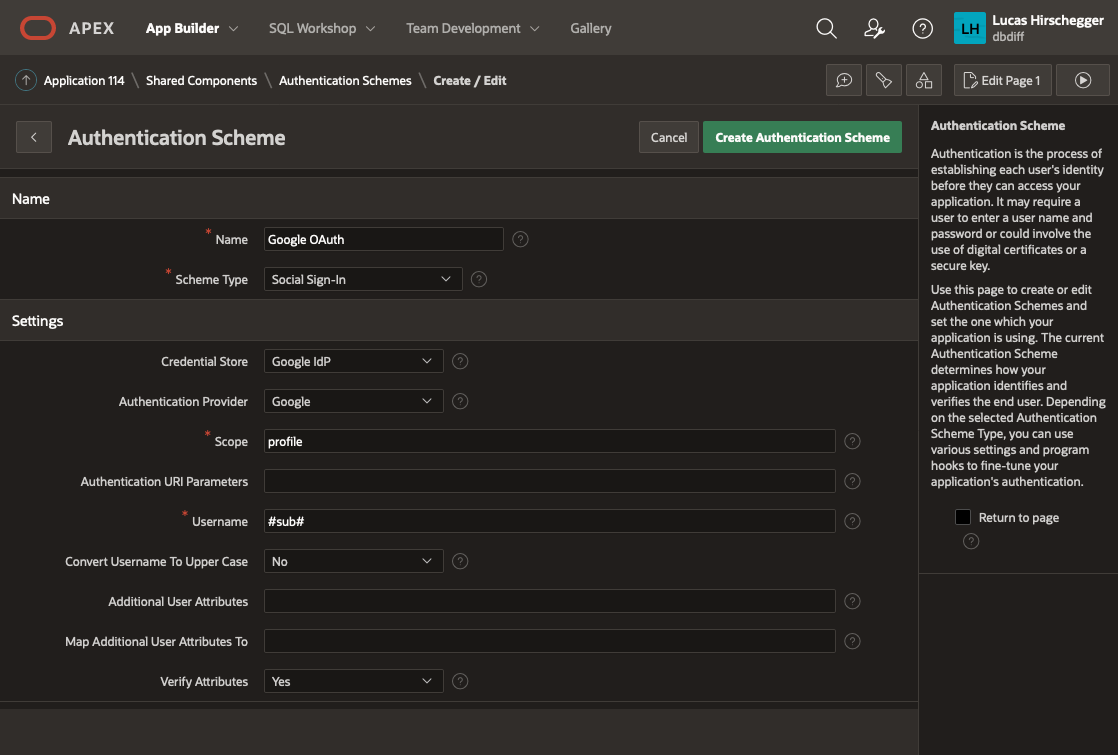
The above page will allow you to specify the following attributes of your Authentication:
- Name – a meaningful name for your scheme
- Scheme Type – select Social Sign-in
- Credential Store – select the one you have just created which is specified at the Workspace level
- Authentication Provider - here select Google. Note that APEX can integrate with any 3rd party IdP as long as they follow the OpenID protocol. Generic Oauth2 providers may be used as well as long as they support OpenId as an inputted scope. In these scenarios you will have to get information on the API end points for authentication / access token and userinfo.
- Scope – we could just use email but enter profile here and I will cover how to extract additional attributes from the JSON that is returned with successful login as an extra feature later on
- Username – here we assign the username to the email address of the Google account user
- Convert Username to upper case. I select yes here so that I lose case sensitivity for usernames but this is just down to what you intend to do with the user name
The discovery URL is not needed here (as it is pre defined by APEX when you select "Google" as then authentication provider, but is worth mentioning. This URL will provide us with JSON that describes this service in detail. You can examine the response by entering it in a browser:
https://accounts.google.com/.well-known/openid-configuration
Of interest to us in particular in the resulting JSON is the section listing claims_supported:
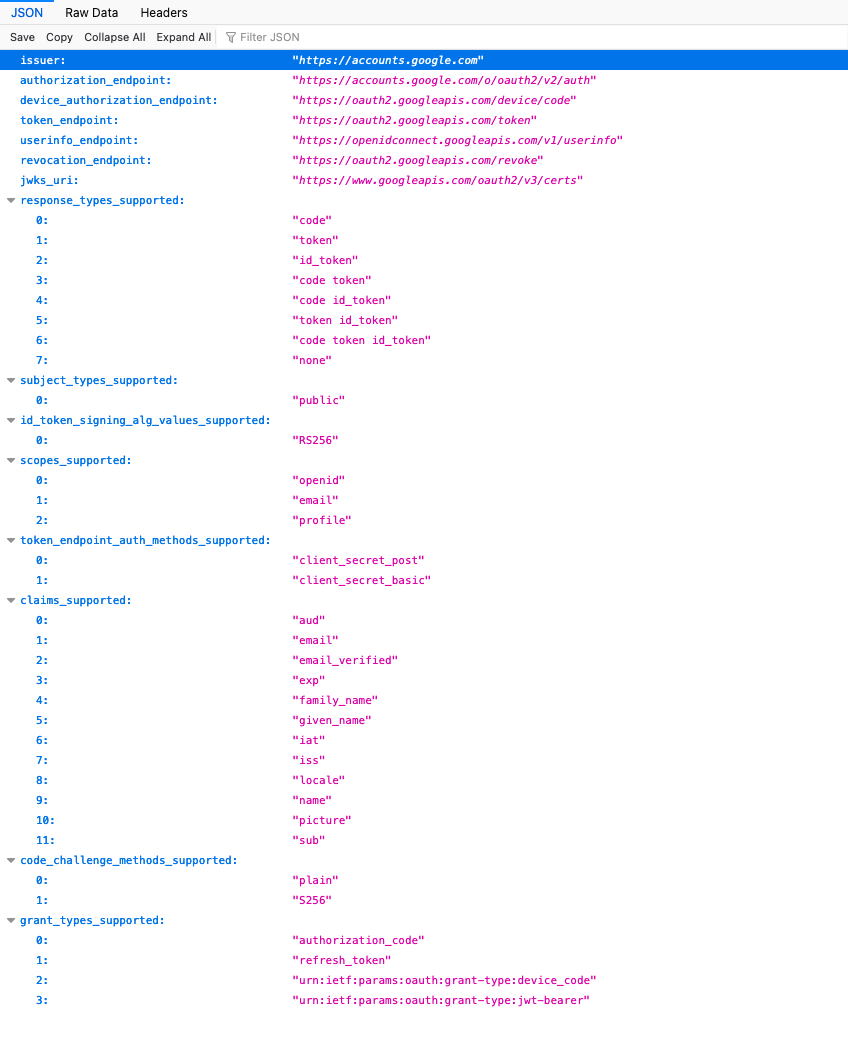
We shall just use email here for the identifier for the username and shall choose the option of making it uppercase in the application.
Click Create and that will complete the creation and switch the default authentication to your Google Authentication.
If you run your application now, you will see no difference. This is because all the pages are currently public and require no authentication or authorizations. We will change page 100 (the Home Page) now to demonstrate how access can be limited to those users you want to authenticate.