Oracle Analytics Cloud November 2022 Update: the New Features, Ranked
The November 2022 Update for Oracle Analytics Cloud came out few days ago and in this blog post I'm going to have a look at all the new features it includes. If you are also interested in a comprehensive list of the defects fixed by the update, please refer to Doc ID 2832903.1.
10. Non-SSL, Kerberos Connections to Hive
Non-SSL connections to a Hive database using Kerberos network authentication protocol are now supported.
9. Enhanced Data Profiling
The random sampling depth and methodology that drives augmented features such as quality insights and semantic recommendations has been improved.
8. Toggle Sample Data Previews in Metadata View
This feature allows users to switch off sample data previews in Metadata view to stop generating the sample values displayed in the Sample Values column and improve their user experience when previews are not required. The toggle switch is displayed at the bottom right of the Metadata view (Figure 1).
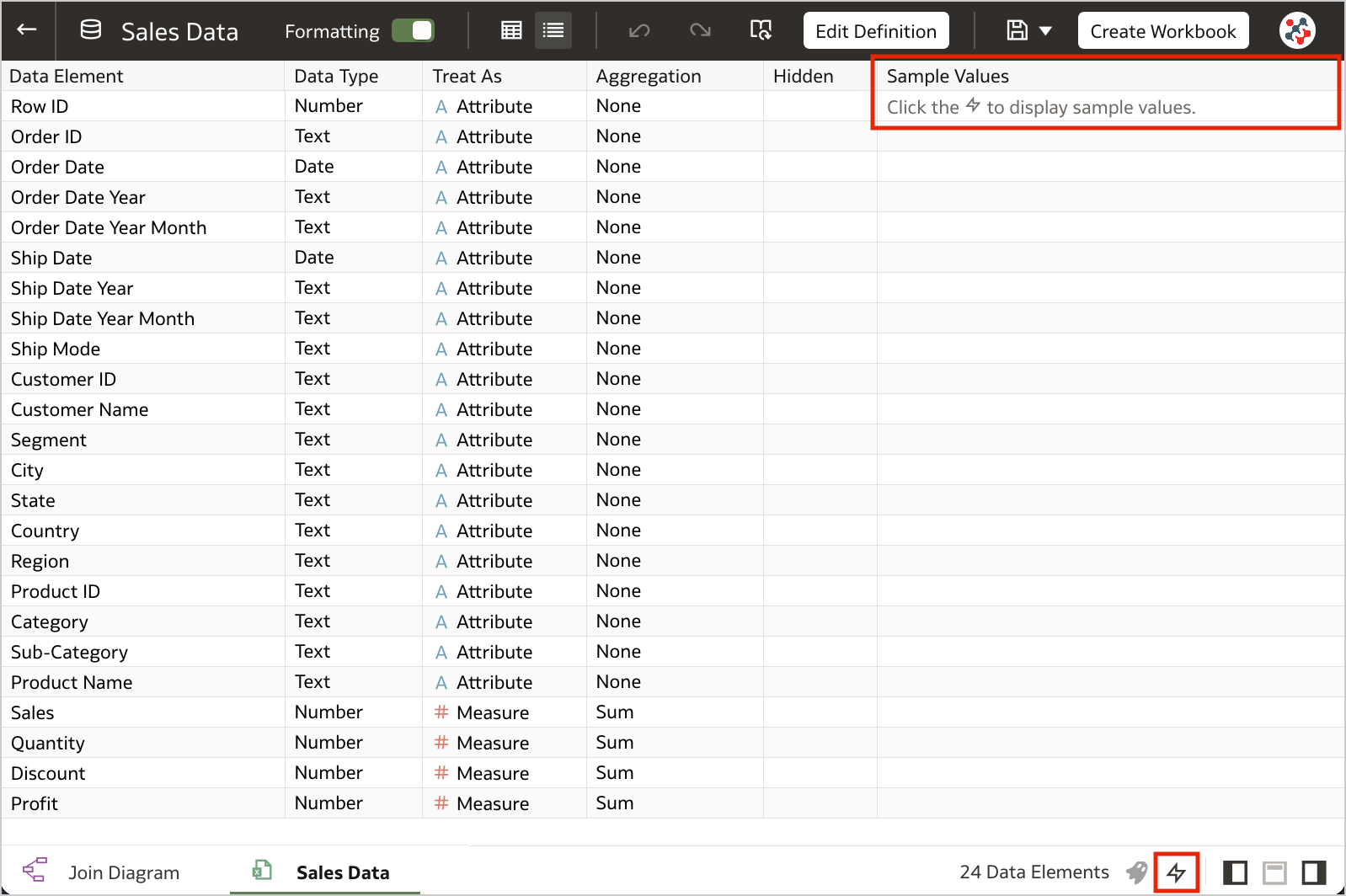
7. Blurred Thumbnails
Workbook thumbnails displayed on the home page are now blurred to protected sensitive data from being exposed to users that don't have the same access as data authors (Figure 2).
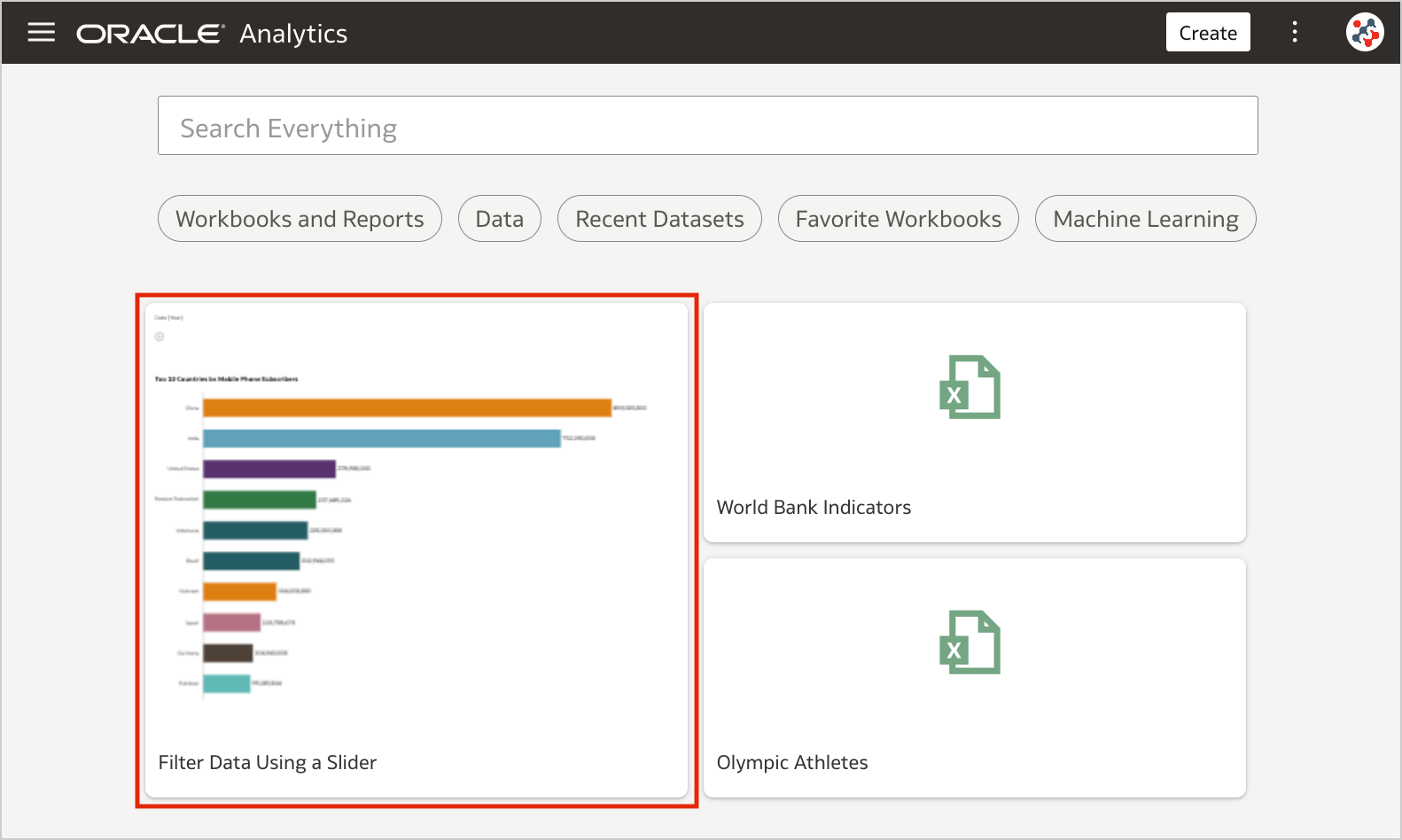
Unfortunately, the blur effect is not sufficient to make performance tile content completely indistinguishable. For additional security, administrators can disable workbook thumbnails altogether by switching off the Save Workbook Thumbnail option in the System Settings section of Data Visualization Console (Figure 3).
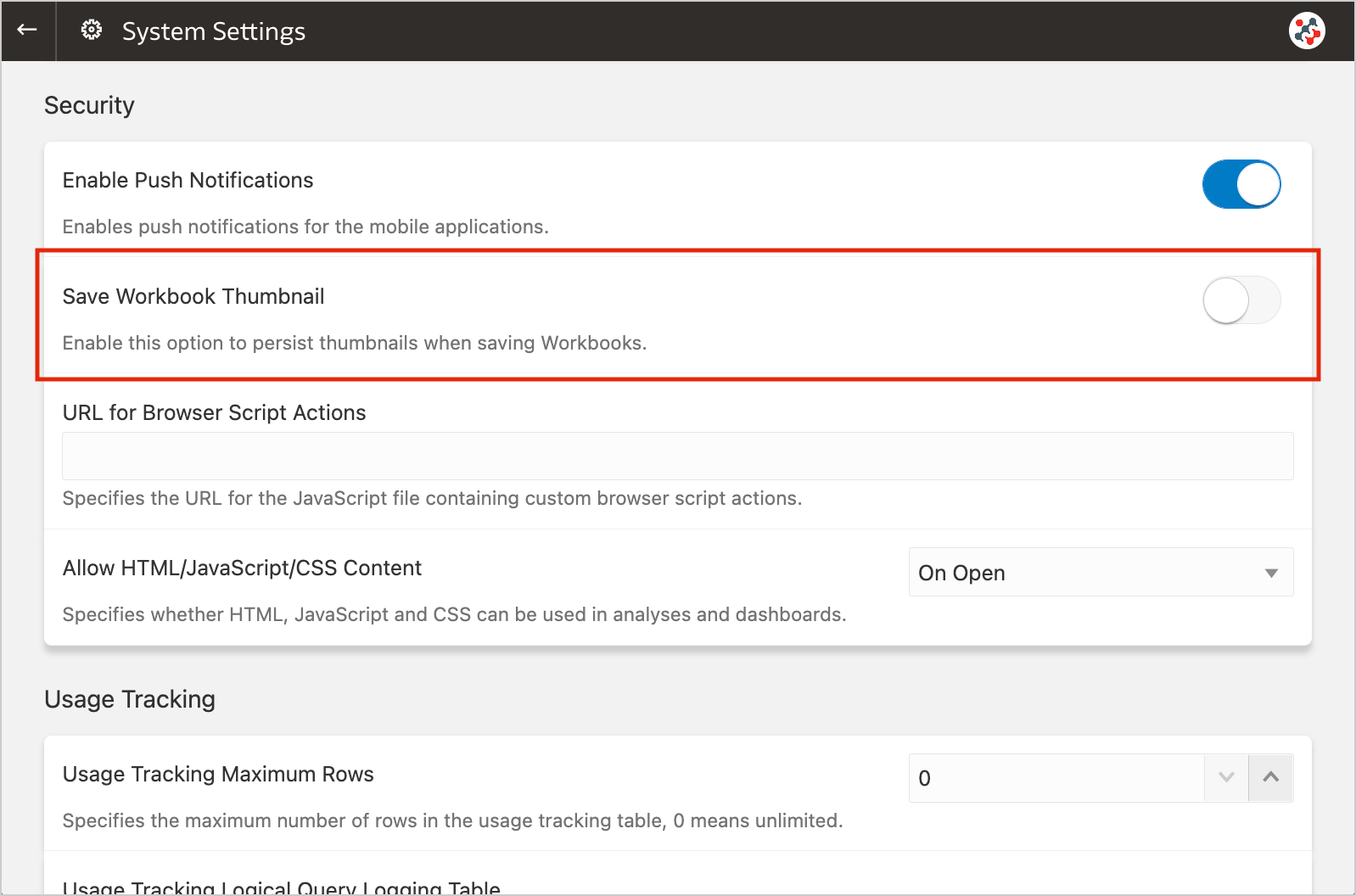
When thumbnails are globally allowed, content authors can show or hide the thumbnail for an individual workbook as required. Click Menu on the workbook toolbar, select Workbook Properties and set Save thumbnails to On or Off (Figure 4).
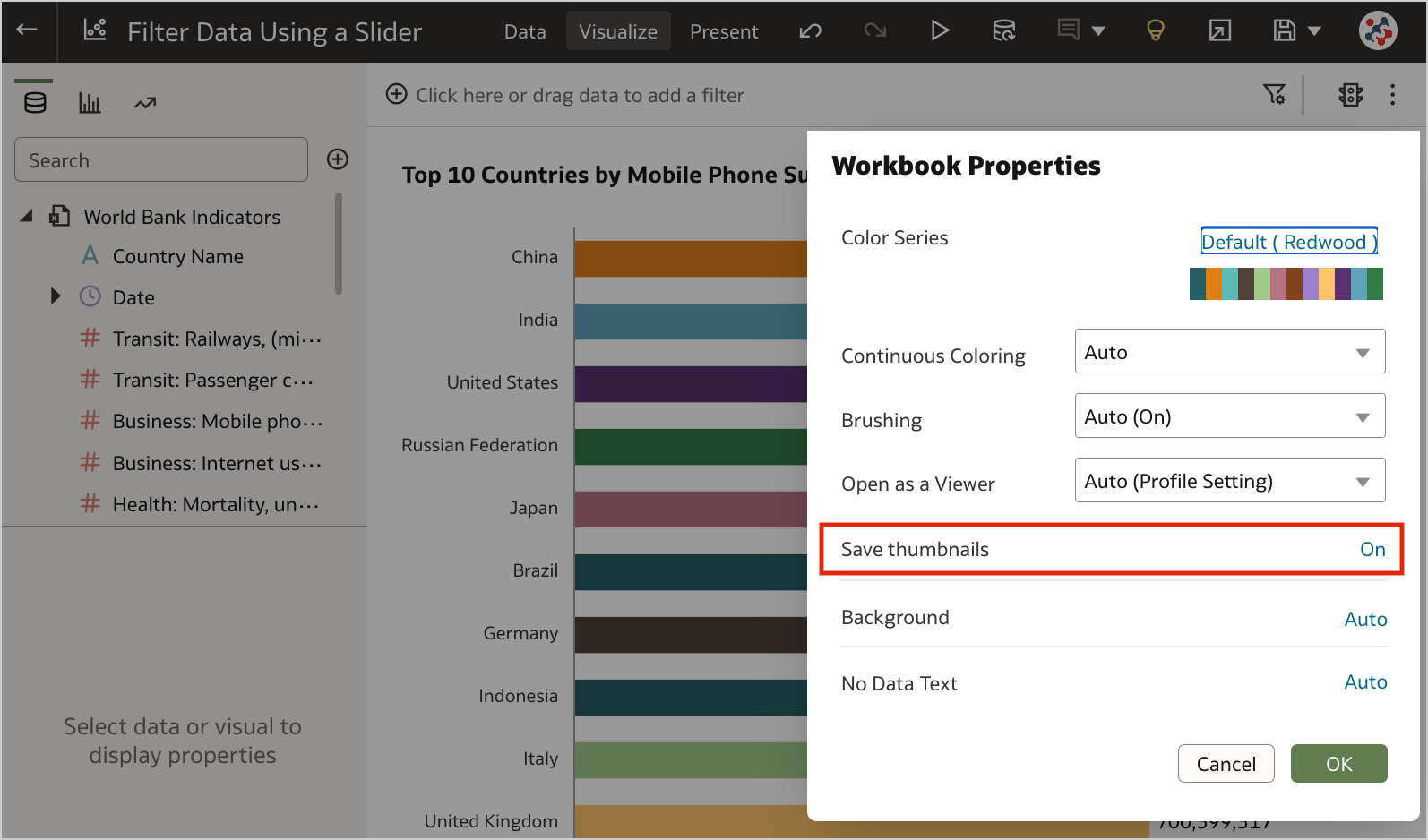
6. Transform Data to Dates More Easily
Unrecognized dates can be transformed more easily using single-click Convert to Date recommendations.
5. Control Filter Interactions from the Filters Bar
When your workbook contains many filters, the Limit Values By icon in the filters bar can be used to toggle between limited filter selection values and unlimited filter selection values (Figure 5).
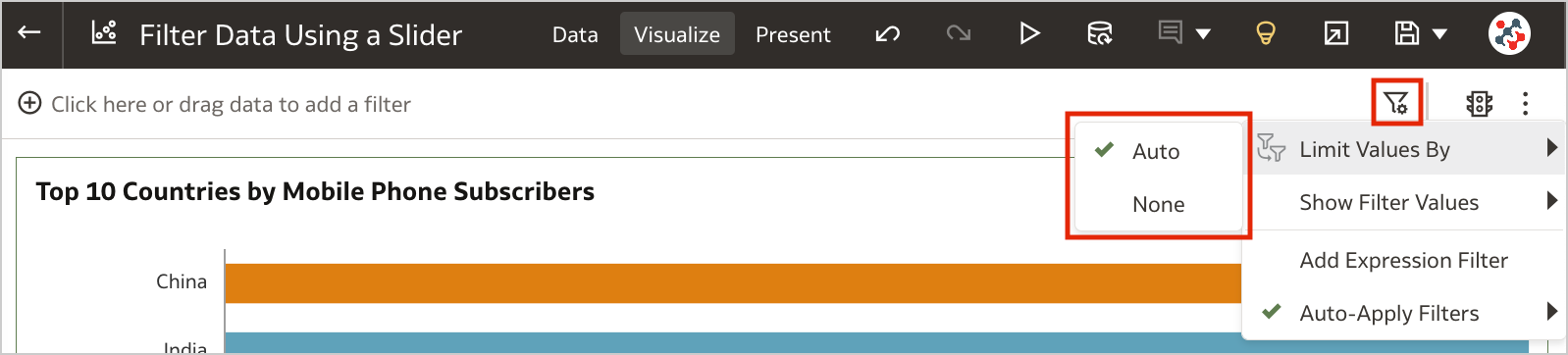
4. Customize the Workbook Header Bar
Authors can show or hide the header bar, and customize it. To customize the header bar color, text, font, and image, go to the Properties panel in the Present page of the workbook and select the Presentation tab (Figure 6). End users can then view the header bar as configured by the author.
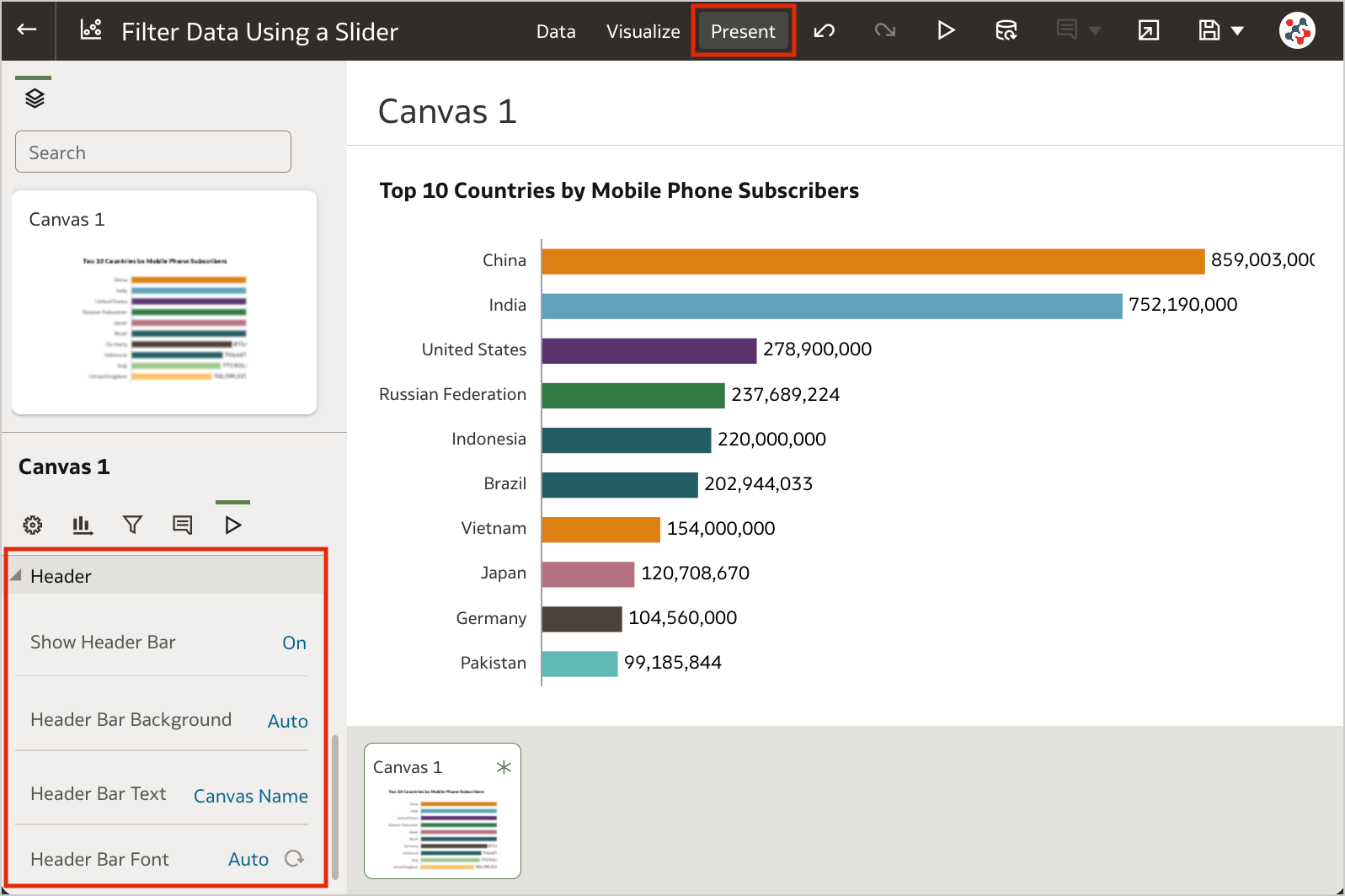
3. Filter Data Using a Slider
Slider dashboard filter can be added to a canvas to animate visualizations and show dynamically how your data changes over a given dimension such as time (Clip 1).
The feature is similar to a section displayed as slider in Analytics, but more powerful: it generates more efficient queries, with a single object you can interact with multiple visualizations at the same time, and it supports tables and pivot tables as well. To make the most of it, I recommend to set up a custom End for Values Axis of your visualizations.
2. Select Columns for Auto Insights
By default, Oracle Analytics profiles all columns in a dataset when it generates insights. Data columns to use for Auto Insights can now be selected by content authors to fine-tune the insights that Oracle Analytics generates for you and focus only on the most useful ones (Figure 7).
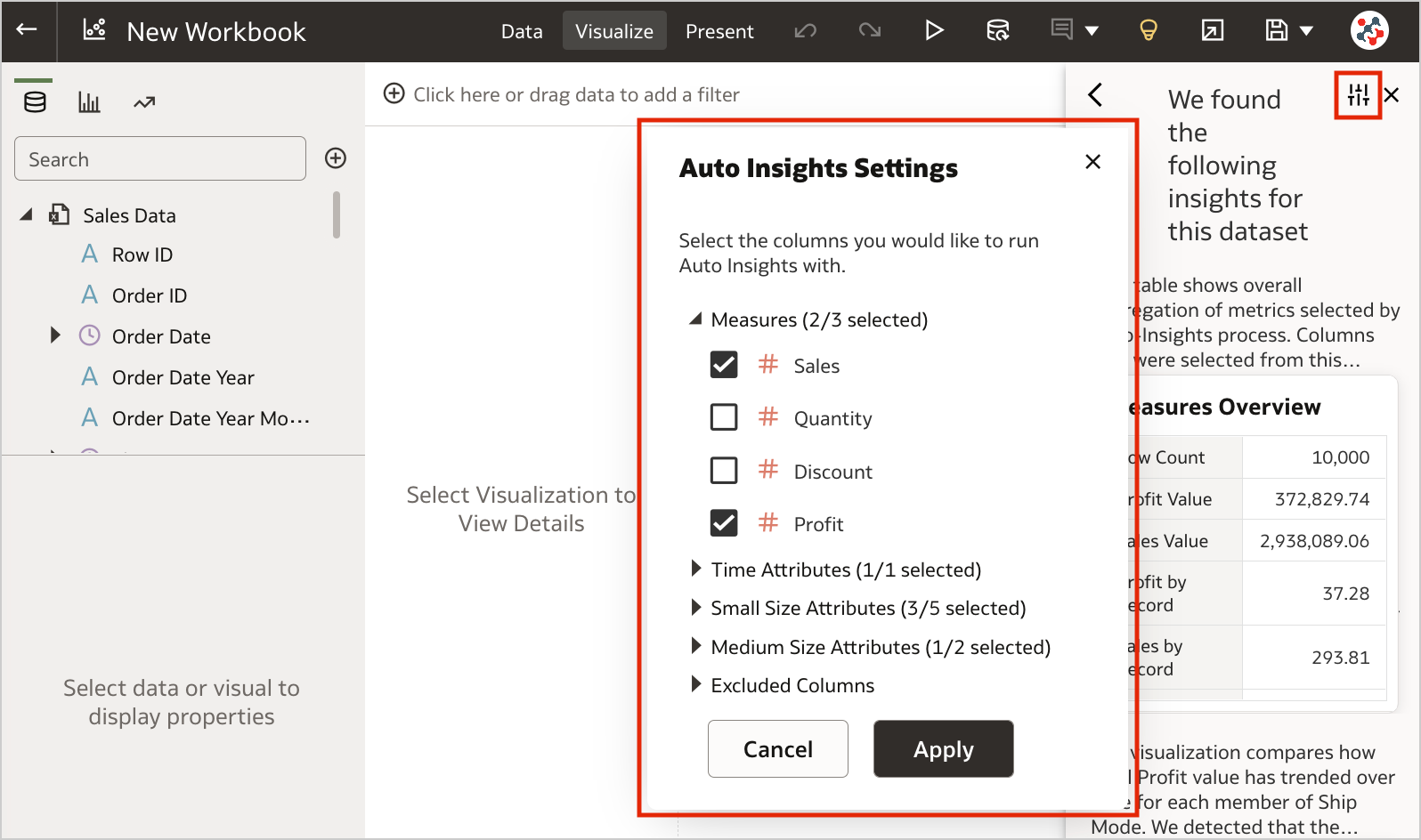
1. Export Table Visualizations to Microsoft Excel
Formatted data from table and pivot table visualizations (up to 25,000 rows) can now be exported to the Microsoft Excel (XLSX) format (Figure 8).
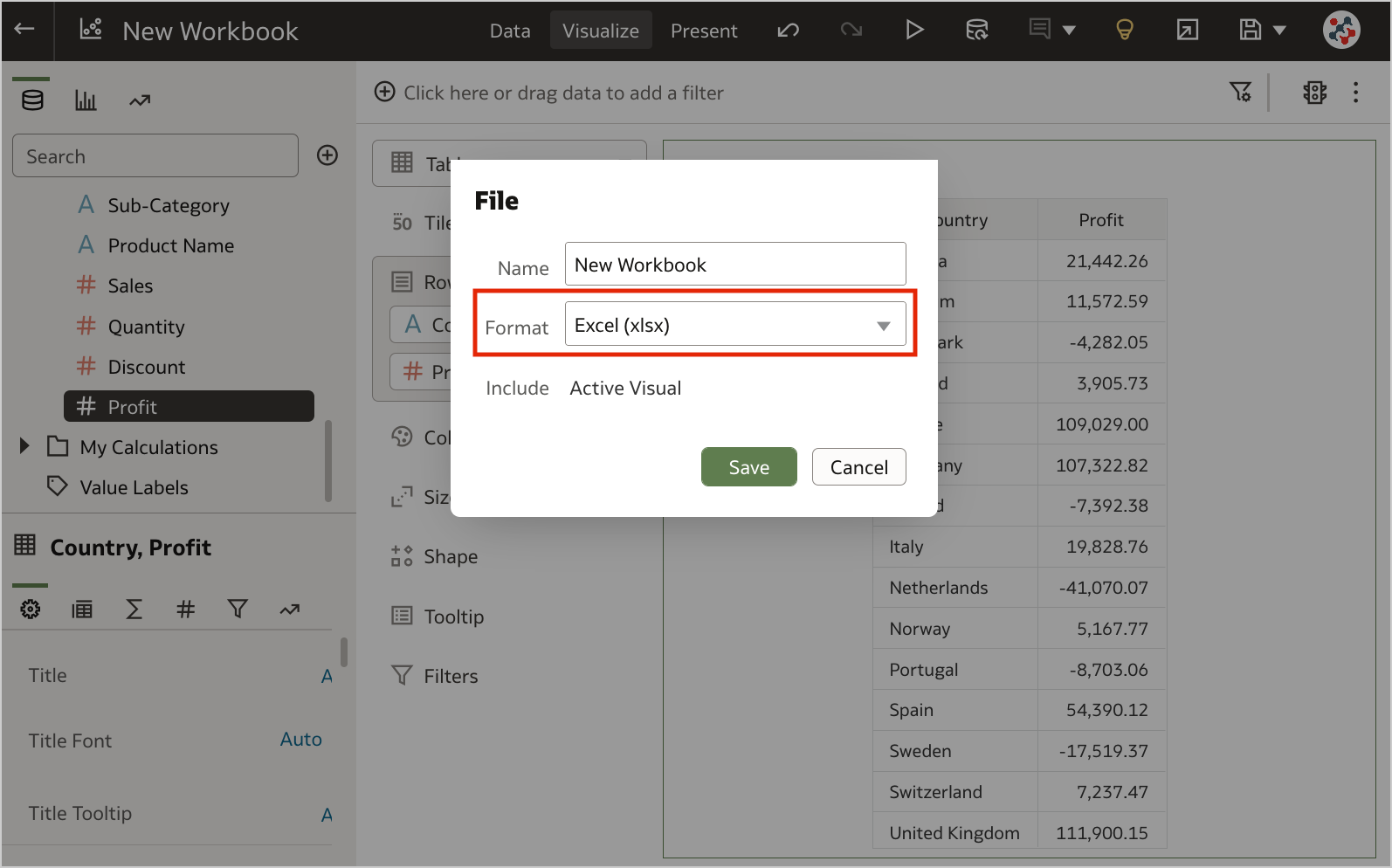
This feature is currently available for preview. Administrators can enable it by switching on the Preview Excel Export option in the System Settings section of Data Visualization Console (Figure 9).
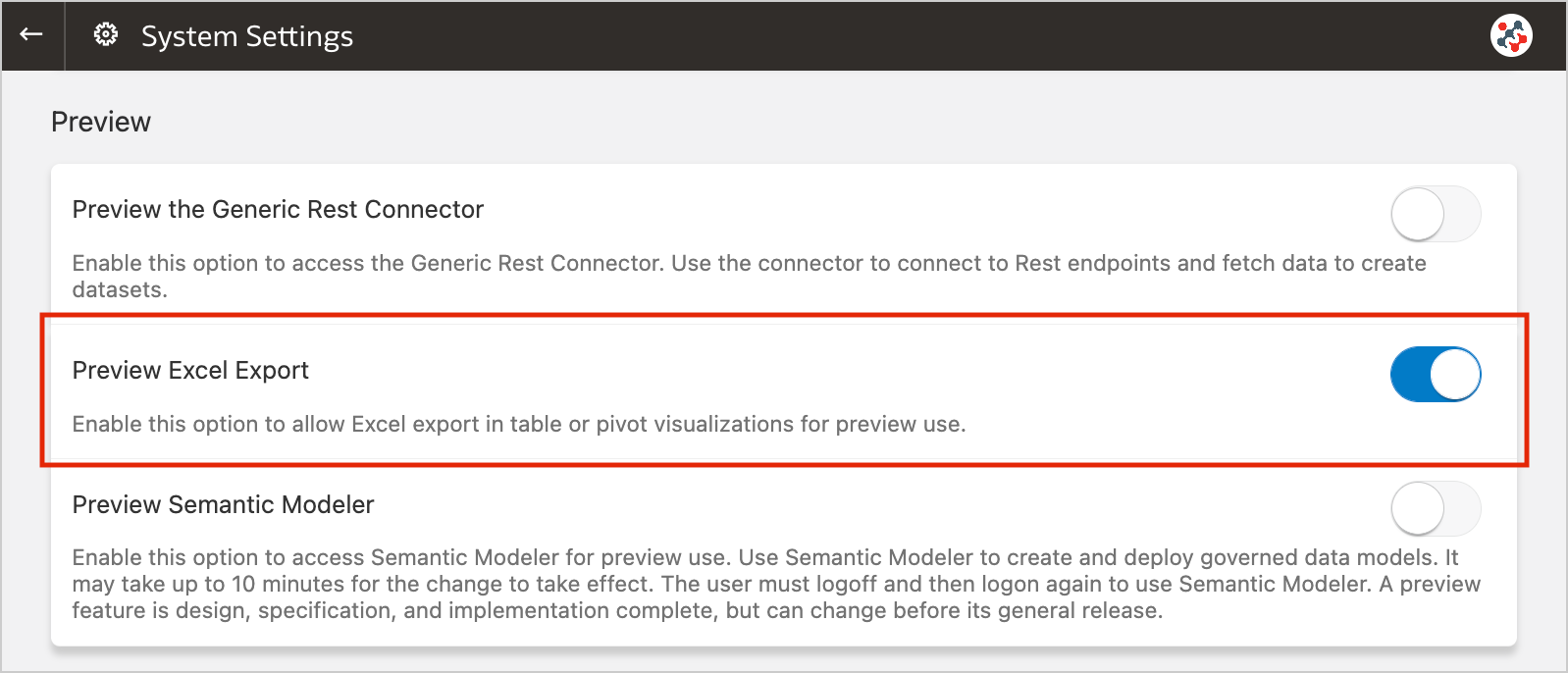
Conclusion
The November 2022 Update includes several new features (and fixes) for Oracle Analytics Cloud that significantly improve Data Visualization and make it more user friendly.
If you are looking into Oracle Analytics Cloud and want to find out more, please do get in touch or DM us on Twitter @rittmanmead. Rittman Mead can help you with a product demo, training and assist within the migration process.
Leveraging Custom Python Scripts in Oracle Analytics Server
Oracle Analytics Server has enabled users to invoke custom Python/R scripts since the end of 2017. Unfortunately, this feature is not yet widely adopted, probably because the official documentation shows only how to upload a custom script, while the details about enabling the feature and embedding the script in XML format are not provided.
In this post, I'm going to illustrate how to leverage custom Python scripts in Oracle Analytics Server to give you greater control and flexibility over specific data processing needs.
Enabling Custom Scripts
The feature to invoke custom scripts is disabled by default and Doc ID 2675894.1 on My Oracle Support explains how to enable it.
Copy the attached updateCustomScriptsProperty.py script to $ORACLE_HOME/bi/modules/oracle.bi.publicscripts/internal/wlst.
Then execute the script using the WebLogic Scripting Tool:
Linux:
$ORACLE_HOME/oracle_common/common/bin/wlst.sh $ORACLE_HOME/bi/modules/oracle.bi.publicscripts/internal/wlst/updateCustomScriptsProperty.py true $DOMAIN_HOME $ORACLE_HOME
Windows:
%ORACLE_HOME%\oracle_common\common\bin\wlst.cmd %ORACLE_HOME%\bi\modules\oracle.bi.publicscripts\internal\wlst\updateCustomScriptsProperty.py true %DOMAIN_HOME% %ORACLE_HOME%
Restart Oracle Analytics Server to enable the feature.
Installing Additional Python Packages
Oracle Analytics Server 6.4 relies on Python 3.5.2 (sigh) which is included out-of-the-box with several packages. You can find them all under $ORACLE_HOME/bi/modules/oracle.bi.dvml/lib/python3.5/site-packages.
Call me paranoid or over-cautious, but to me it makes sense not to play around with the out-of-the-box version put in place by the installation. To avoid this, if any additional packages are required, I choose to firstly install another copy of the same Python version (3.5.2) in another location on the server - this way, I know I can add to or make changes without possibly affecting any other standard functionality that uses the out-of-the-box version.
Installing Python 3.5.2 on Linux in 2022 could be a bit tricky since the Python official website does not host the installers for older versions, but only the source code.
First of all download the source code for Python 3.5.2.
$ wget https://www.python.org/ftp/python/3.5.2/Python-3.5.2.tgz
Now extract the downloaded package.
$ sudo tar xzf Python-3.5.2.tgz
Compile the source code on your system using altinstall.
$ cd Python-3.5.2
$ sudo ./configure
$ sudo make altinstall
Then install all required packages using pip. My example needs langdetect and in order to make it work correctly with Python 3.5.2 I decided to install an older version of it (1.0.7). You should always verify which versions of packages used in your code are compatible with Python 3.5.2 and install them explicitly, otherwise pip will automatically pick the latest ones (which may not be compatible).
$ sudo pip3.5 install langdetect==1.0.7
Edit the obis.properties file located under $DOMAIN_HOME/config/fmwconfig/bienv/OBIS, set the PYTHONPATH variable to ensure the packages can be found by Oracle Analytics Server, and restart the services.
PYTHONPATH=$ORACLE_HOME/bi/bifoundation/advanced_analytics/python_scripts:/usr/local/lib/python3.5/site-packages
export PYTHONPATH
Anatomy of a Custom Python Script
To be able to use a custom Python script with Oracle Analytics Server, we need to embed it in a simple pre-defined XML format.
The XML must contain one root element <script> that is the parent of all other elements:
<?xml version="1.0" encoding="UTF-8"?>
<script>
...
</script>
The <scriptname> element indicates the name of your script:
<scriptname>py.DetectLanguage</scriptname>
According to the documentation, <scriptlabel> should indicate the name of the script as visible for end users, but it seems to be ignored once the script has been uploaded to Oracle Analytics Server. However, if you don't include this element in the XML you will get an error notification while uploading the script.
<scriptlabel>Detect Language (py)</scriptlabel>
<target> refers to the type of script that you are embedding in the XML:
<target>python</target>
In order to use the script in data flows, it's mandatory to include the <type> element and set it to execute_script:
<type>execute_script</type>
<scriptdescription> is straightforward to understand and provides a description of the script as explained by its developer. You can also specify the version of the script in the <version> element.
<scriptdescription>
<![CDATA[
Determine the language of a piece of text.
]]>
</scriptdescription>
<version>v1</version>
The <outputs> element lists the outputs returned by the script. In the example, the script returns one column called language. <displayName> and <datatype> elements refer to the name displayed in the user interface and the data type of the outputs.
<outputs>
<column>
<name>language</name>
<displayName>language</displayName>
<datatype>varchar(100)</datatype>
</column>
</outputs>
The <options> element indicates the input parameters to the script. There is also a special parameter includeInputColumns which lets users choose whether to append output columns to the input dataset and return, or just return the output columns. In the example, the script requires one column input (text) and always append the output column (language) to the input dataset.
<options>
<option>
<name>text</name>
<displayName>Text</displayName>
<type>column</type>
<required>true</required>
<description>The input column for detecting the language</description>
<domain></domain>
<ui-config></ui-config>
</option>
<option>
<name>includeInputColumns</name>
<displayName>Include Input Columns</displayName>
<value>true</value>
<required>false</required>
<type>boolean</type>
<hidden>true</hidden>
<ui-config></ui-config>
</option>
</options>
And lastly, the <scriptcontent> element must contain the Python code. You have to import all required packages and implement your data transformation logic in the obi_execute_script function:
- The data parameter provides access to the input dataset in a Pandas DataFrame structure.
- The args parameter provides access to the input parameters as defined in the <options> XML element.
- The function must return a Pandas DataFrame structure.
- Oracle Analytics Server will automatically execute this function when you invoke the custom script.
<scriptcontent><![CDATA[
import pandas as pd
from langdetect import detect, DetectorFactory
def obi_execute_script(data, columnMetadata, args):
language_array = []
DetectorFactory.seed = 0
for value in data[args['Text']]:
language_array.append(detect(value))
data.insert(loc=0, column='language', value=language_array)
return data
]]></scriptcontent>
In the example above, the values in the input column are analyzed to detect their language using the langdetect package, the results are then collected into an array and returned alongside the input dataset. The source code is attached below, feel free to use it, but remember to install all required packages first!
Custom Python Scripts in Action
Once wrapped into XML, administrators can upload custom scripts into Oracle Analytics Server. Once uploaded they can be shared and executed by other users.
In the Home page, click on the Create button and select the Script option.
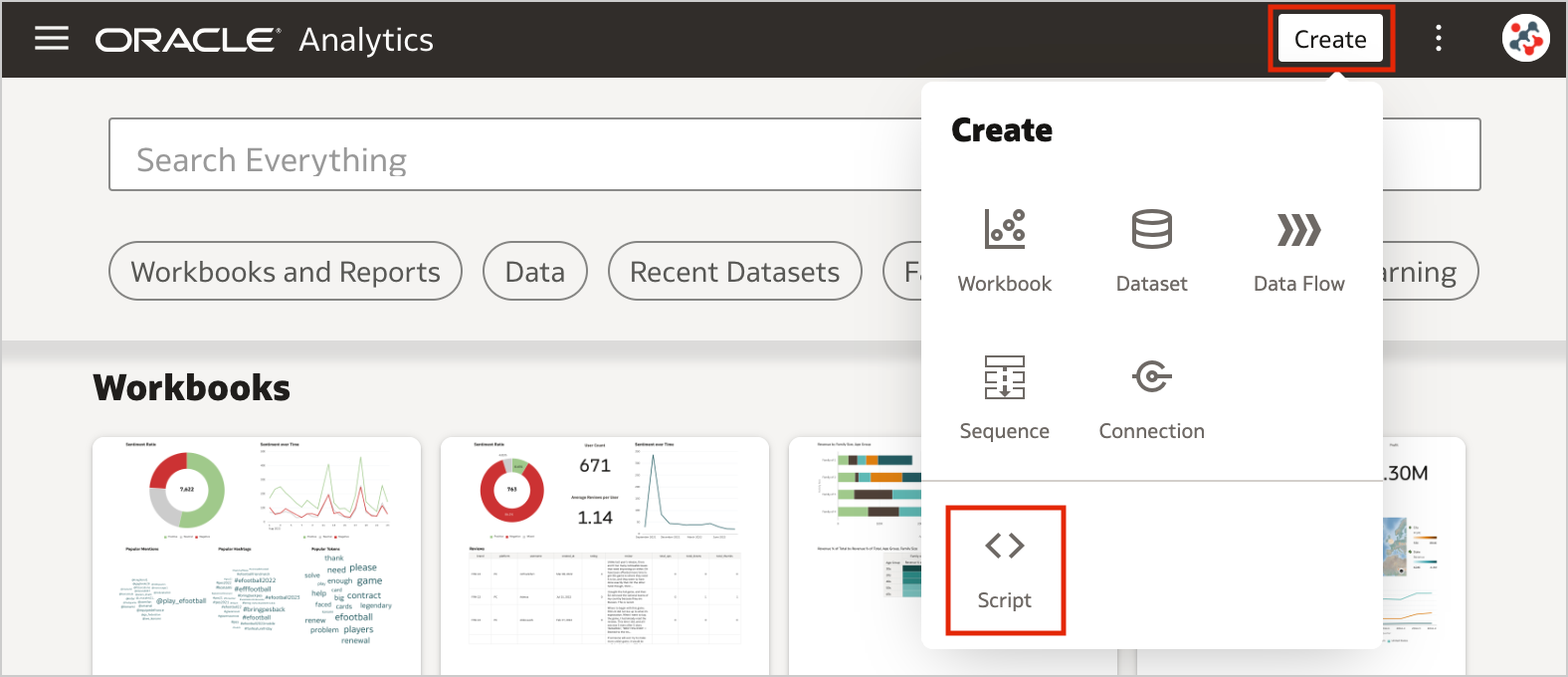
Drag and drop your custom script to the Add Script dialog, then click OK.
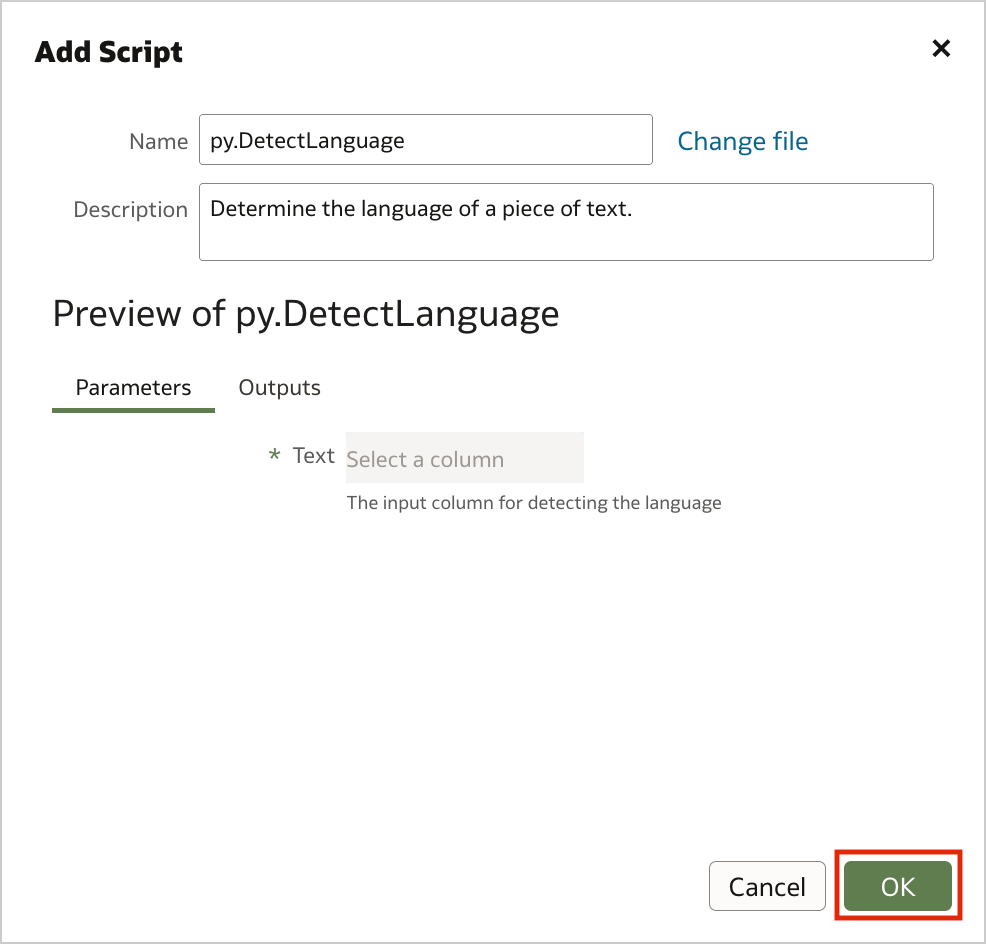
The uploaded custom script is now available for use and displayed in the Scripts tab on the Machine Learning page.
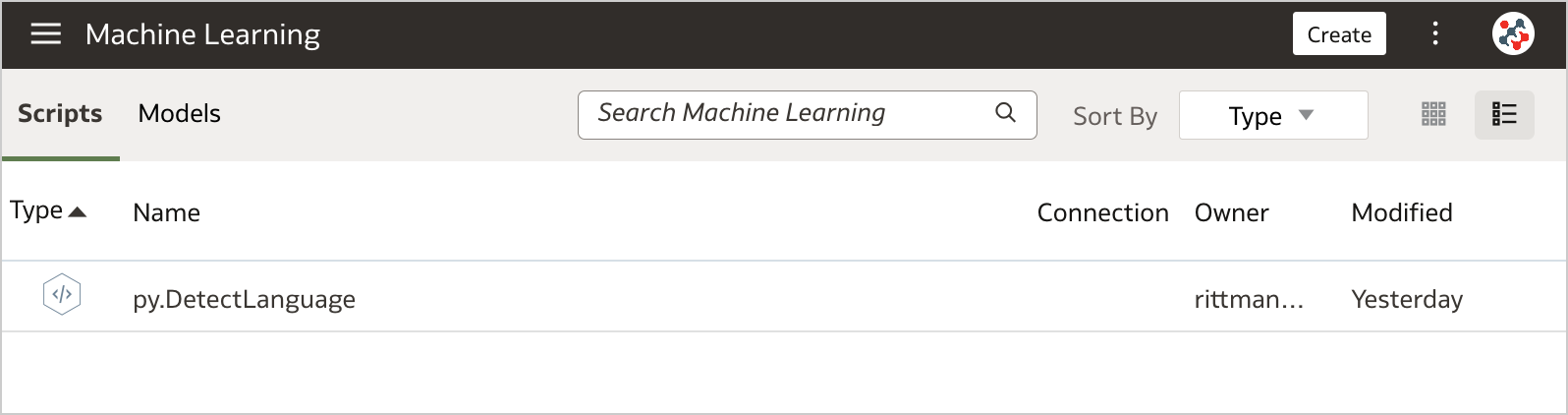
To invoke the script from a data flow you have to include the Add Custom Script step.
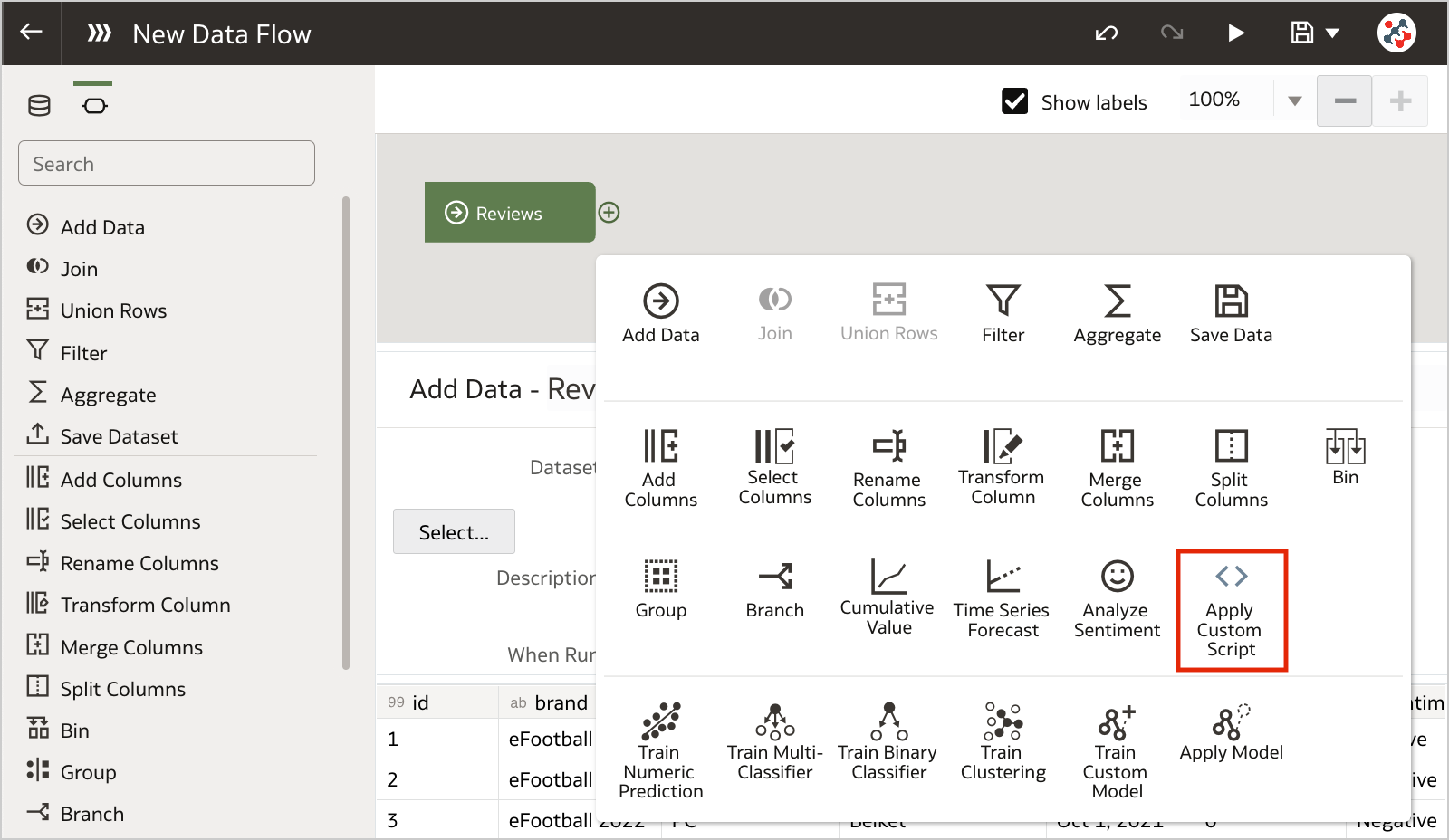
Select the script that you want to execute, and click OK.
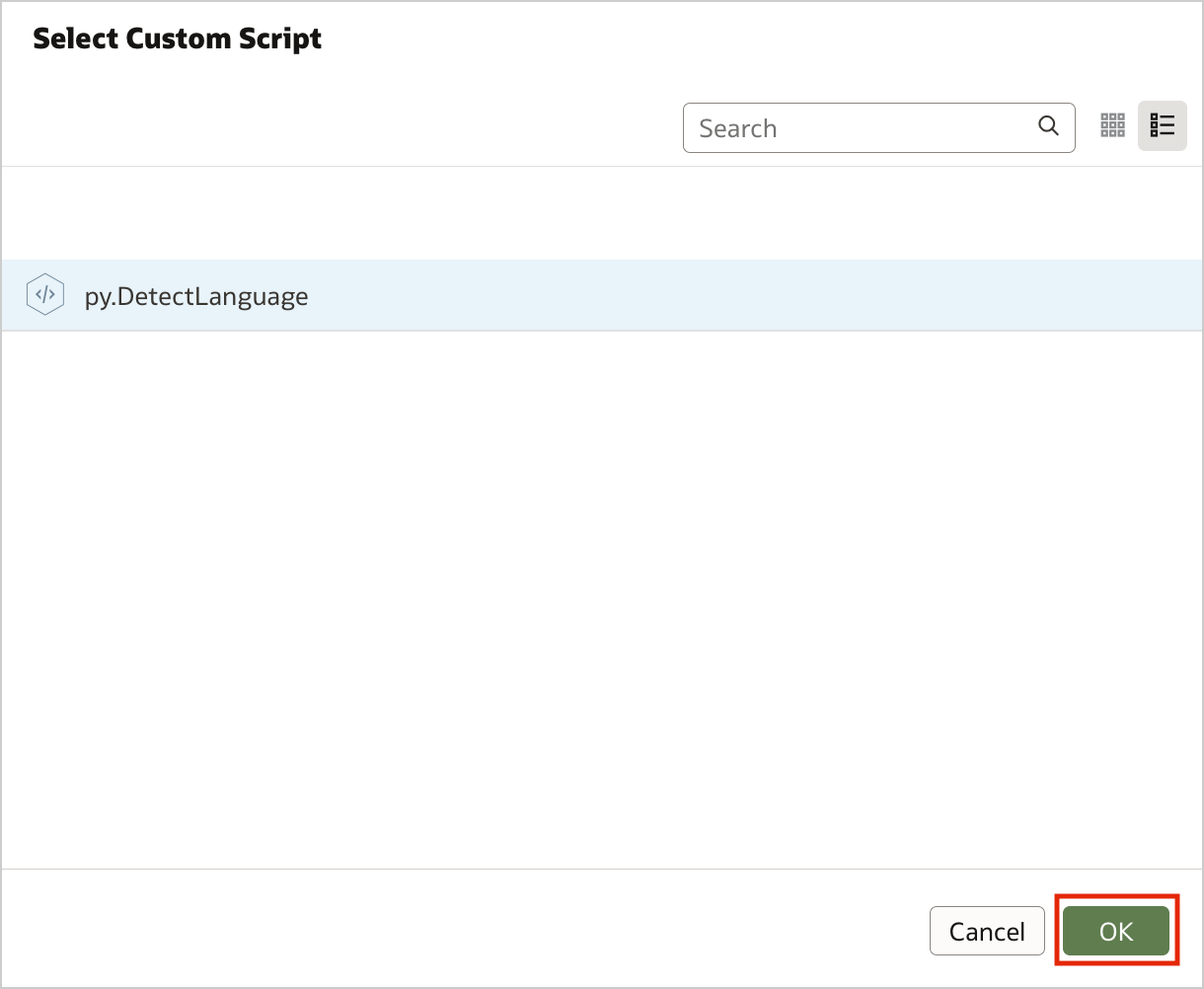
Configure the step by specifying any required input and the outputs that you want to include into the result set. In the example, I chose to detect the language for the review column.
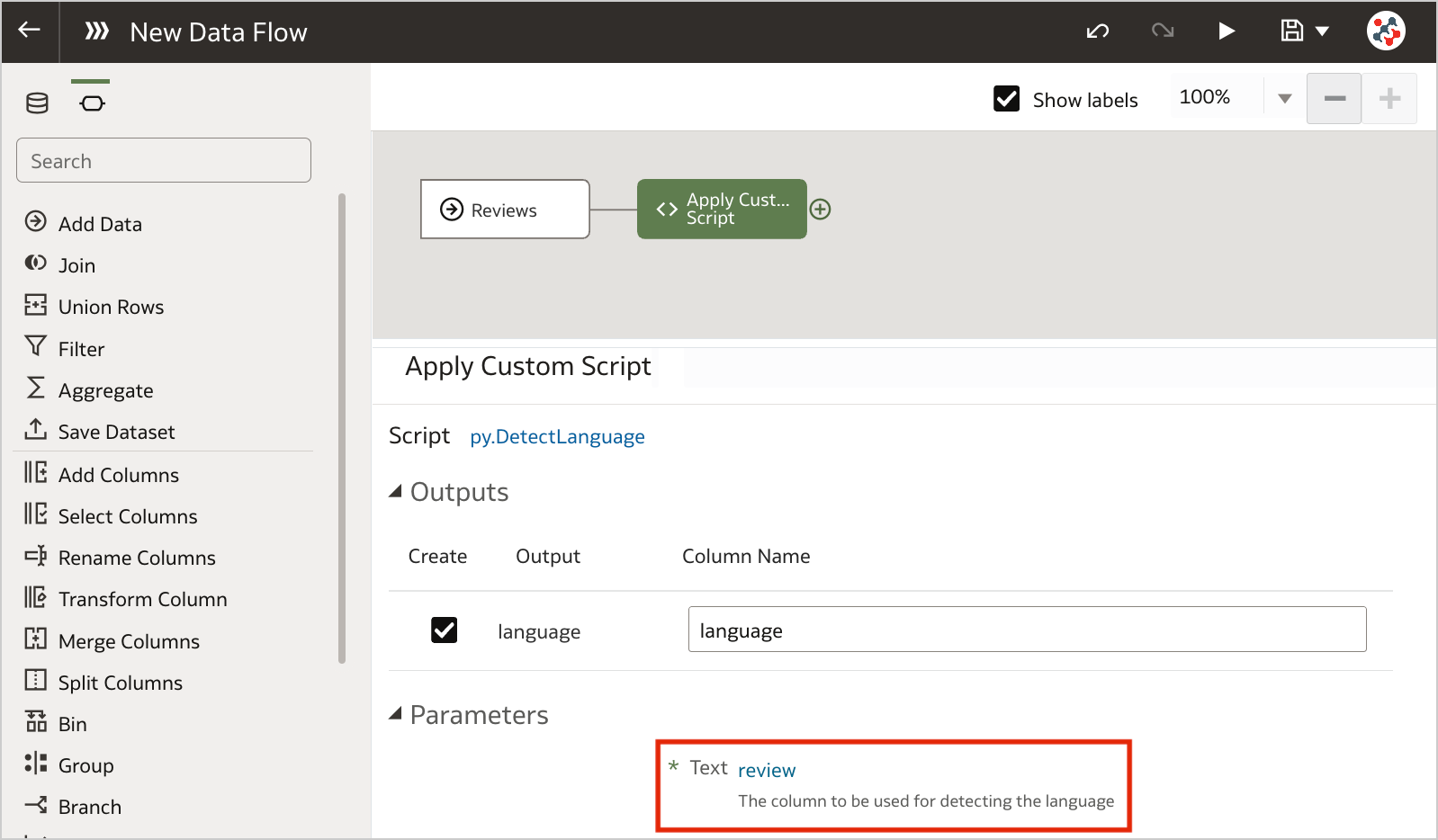
Save the output data by adding the Save Data step and run the data flow to execute the custom script.
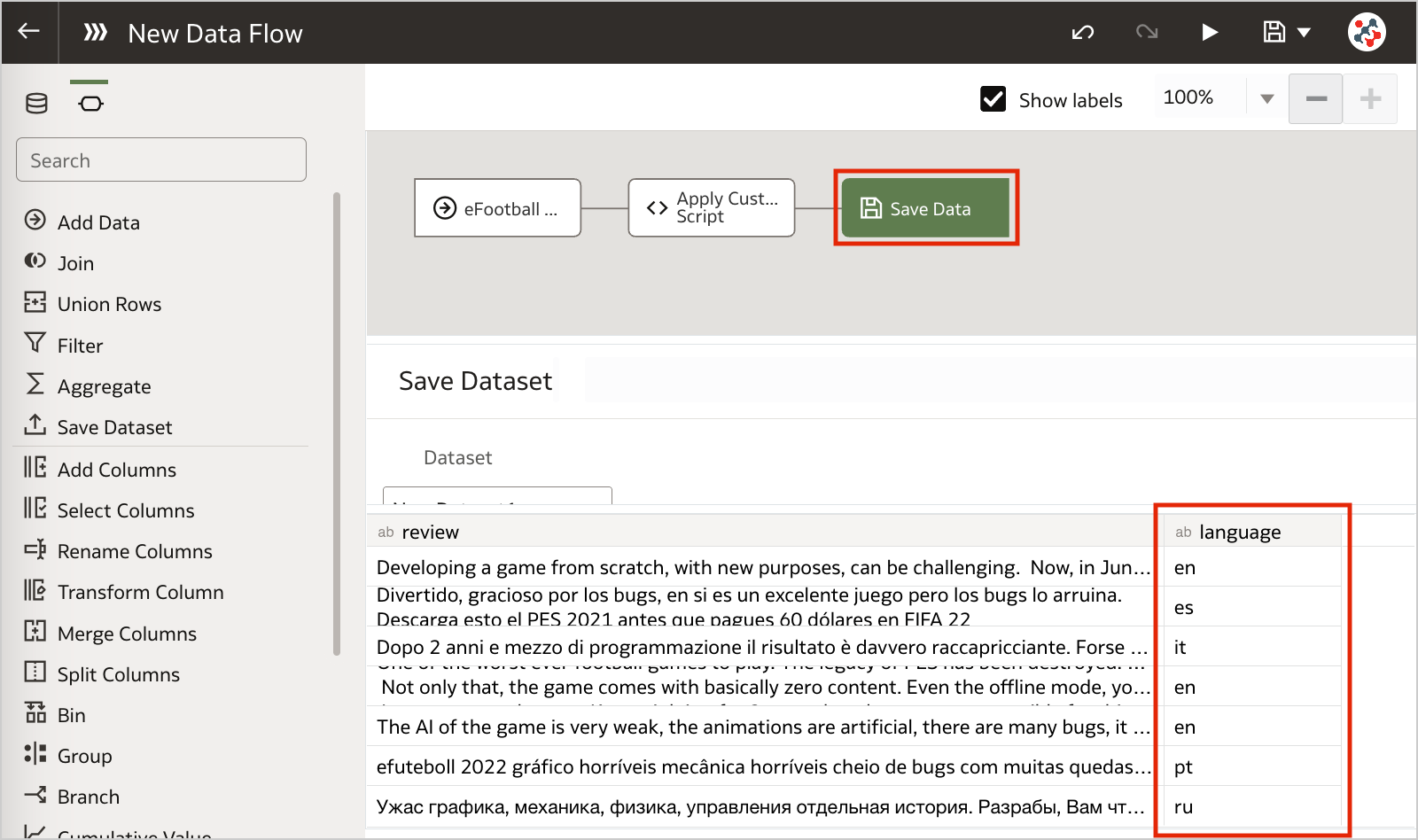
Conclusion
Business analysts and end-users often want greater control when performing data preparation tasks. In this context, leveraging custom Python/R scripts into Oracle Analytics Server can give you full control and flexibility over specific data processing needs.
If you are looking into leveraging custom Python/R scripts into Oracle Analytics Server and want to find out more, please do get in touch or DM us on Twitter @rittmanmead. Rittman Mead can help you with a product demo, training and assist within the development process.
Rittman Mead – de Novo Partnership

26th October 2022
On behalf of Rittman Mead I am delighted to announce the market disrupting partnership of de Novo - Rittman Mead.
Oracle is a large investment for any organisation and as such it needs to be done correctly. By combining forces this new partnership gives clients access to on shore specialists across Finance, HR, Data & Analytics.
I’ve been in the Oracle market in one way or another for 16 years and one story has remained consistent throughout. When a client has a large transformation programme they usually engage with a massive SI that promise the world, but in most cases fail to deliver on that promise.
In fact in the mid naughties it was more common to find a project running at 50% over budget and six months past the deadline than one that actually went as planned.
There’s always been a hunger to get true experts working in collaboration and we see this as the start of that journey.
Meet the Team
Rittman Mead
We’ve been a leader in the Oracle BI, Data & Analytics world for 15 years and believe successful organisations see data as an opportunity, not an overhead. Over that time, we’ve helped companies do exactly that, by partnering with companies we enable them to produce understandable content from their data and drive meaningful business change.
de Novo
A real A team in the world of Oracle Finance and HR. With hundreds of hours of transformation work under their belts for clients from SMB to Enterprise. de Novo not only understand the practicalities of a great Oracle transformation they have the departmental knowledge to make sure your Finance and HR teams are listened to and included in the creation of world class solution.
With the uncertainties facing everyone over the next 12 to 18 months there has never been a more business critical time to do projects correctly from day one, Rittman Mead and de Novo not only offer a true best in breed team, built with the top 10% of consultants in their respective niches. They deliver a real client - consultancy partnership.
For additional information please feel free to contact;
Oliver Matthews - Business Development - Rittman Mead
oliver.matthews@rittmanmead.com
+44 (0) 7766060749
Michelle Clelland - Marketing Experience Lead - de Novo Solutions
michelle.celland@de-novo-solutions.com
+44 (0) 1633492042
Commute Vs Energy App
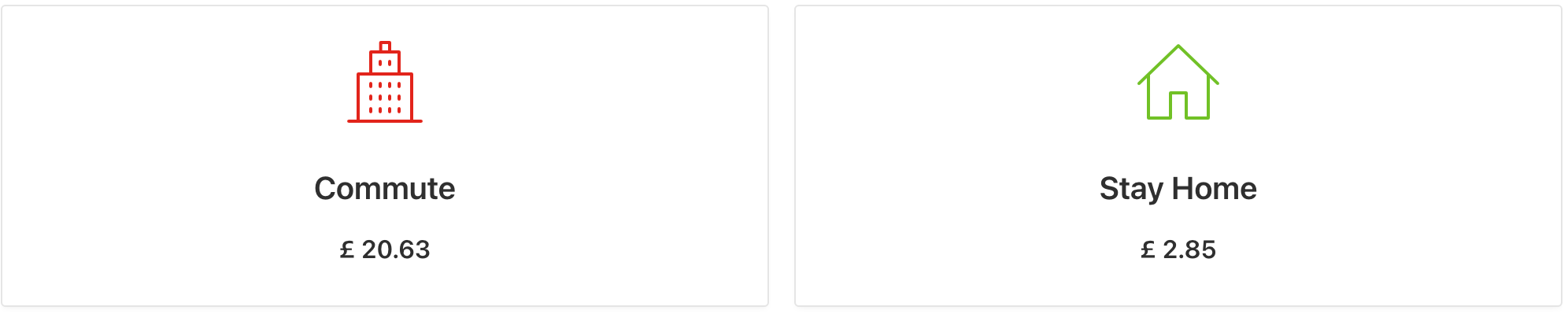
23% of UK workers are considering ditching WFH to save on heating bills!!! Was the title of a news article that appeared on my phone at the end of August.
Back then, and I say back then because the rate at which things are changing in the UK make a month feel more like a years worth of policy and financial worry. The fear was, energy prices could continue to rise every quarter until we’d all be selling a kidney every time we wanted a bath.
For a second it made me think this could actually have some legs.
Then it made me wonder. What about the cost of commuting?
For instance I used to live in Tunbridge Wells and I remember clearly the train season ticket being £6000 to get to London. So, would 10 back to the office hours including commuting actually save me anything or would it just cost me more?
The answer in that scenario was obviously a resounding no! Going to the office was the financially un responsible move.
But there must be some situations where going back to the office makes sense. 23% of UK workers can’t be completely mad. Can they? What home to work distance makes going to the office to get warm economically viable? How much of a difference does the way you get to work make? For instance walking is free, a 6.2 ltr V8 is not so free.
There were too many factors to fit onto a single A4 sheet of paper, so as is the case in many businesses, I released my Excel abilities (predominately the =sum() one). I produced a good data set, with the ability to recalculate based on the miles to office, chosen vehicle, lunch and parking if appropriate.
For me commuting failed to win the economically viable game even after I removed all vehicle related expenses other than fuel. But it was fun and I wanted to share it.
Luckily for me we have a Lucas at Rittman Mead.
For the uninitiated a Lucas is an APEX guru able to spin up even the most random of concepts into a well designed, functional APEX application. Over a few days of listening to Oliver the Sales / Amateur Product Owner explain how he wanted a slidey thing for this and a button for that. Lucas was able to produce my vision in a way others could enjoy.
So here it is the Commute vs Energy application brought to you by the APEX Team at Rittman Mead.
https://commute.ritt.md/ords/r/energy_tool/calc/home?session=404835286670167
Disclamer
The Commute vs Energy app is the brain child of a non technical Sales man who’d had a lot of coffee. So while it does give you a calculated answer based on your options it is in no way an exhausted analysis of every possible penny involved in your WFH or not decision. It’s a fun slightly informative tool for your pleasure.
How to Sense-Check Your Data Science Findings
Introduction
One of the most common pitfalls in data science is investing too much time investigating a dead-end. It happens to the best of us; you're convinced that you've found something amazing and profound that will revolutionise the client's business intelligence...And then, in an awkward presentation to a senior stakeholder, somebody points out that your figures are impossible and somewhere along the way you've accidentally taken a sum instead of an average. Here are a few tricks you can and should use to make this kind of embarrassment less likely.
Visualise Early and Often
The sooner you plot your data, the quicker you will notice anomalies. Plot as many variables as you have time for, just to familiarise yourself with the data and check that nothing is seriously wrong. Some useful questions to ask yourself are:
• Is anything about these data literally impossible? E.g., is there an 'age' column where someone is over 200 years old?
• Are there any outliers? Sometimes data that has not been adequately quality-checked will have outliers due to things like decimal-point errors.
• Are there duplicates? Sometimes this is to be expected, but you should keep it in mind in case you end up double-counting duplicate entries.
If It's Interesting, It's Suspicious
I once worked on a project that found that social deprivation scores negatively correlated with mental health outcomes, i.e., more deprived groups had better mental health scores. This was exactly the kind of surprising result that would have made for a great story, with the unfortunate caveat that it wasn't at all true.
It turned out that I had mis-coded a variable; all my zeroes were ones, and all my ones were zeroes. Fortunately I spotted this before the time came to present my findings, but this story illustrates an important lesson:
Findings that are interesting and findings that are erroneous share a common property: both are surprising.
Talk to Subject-Matter Experts
'Ah, this is impossible- this number can never go above ten' is one of the most heart-breaking sentences I've ever heard in my career.
It's often the case that someone more familiar with the data will be able to spot an error that an analyst recently brought onto a project will miss. It is essential to consult subject-matter experts often to get their eyes on your findings.
Check for Missing Data
There is a legendary story in data collection about Survivorship Bias. In WWII, a group called the Statistical Research Group was looking to minimise bombers lost to enemy gunfire. A statistician name Abraham Wald made the shrewd observation that reinforcements should be added to the sections of planes that returned from missions without bullet holes in them, rather than- as intuition might suggestion- the parts riddled with bullets. This was because those planes were the ones that returned safely, and so the parts that were hit were not essential to keeping the pilot alive.
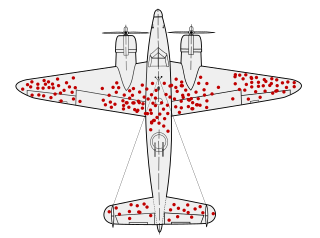
Missing data can poison a project's findings. There are a lot of reasons data could be missing, some more pernicious than others. If data is missing not-at-random (MNAR) it may obscure a systematic issue with data collection. It's very important to keep this in mind with something like survey data, where you should expect people who abandon the survey to be qualitatively different to people who complete it.
Understand Where the Data Came From and Why
Did you assemble this dataset yourself? If so, how recently did you assemble it, and do you still remember all of the filters and transformations you applied to get it in its current form? If someone else assembled it, did they document what steps they took and are they still around to ask? Sometimes a dataset will be constructed with a certain use case in mind different from your use case, and without knowing the logic that went into making it you run the risk of building atop a foundation of errors.
Beware the Perils of Time Travel
This one isn't a risk for all kinds of data, but it's a big risk for data with a date component- especially time series data. In brief, there is a phenomenon called Data Leakage wherein a model will be built such that it unintentionally cheats by using future data to predict the past. This trick only works when looking retrospectively because in the real world, we cannot see the future. Data leakage is a big enough topic to deserve its own article, but just be aware that you should look it up before building any machine learning models.
Conclusion
It is impossible to come up with a fully-general guard against basic errors, and kidding yourself into thinking you have one will only leave you more vulnerable to them. Even if you do everything right, some things are going to slip you by. I encourage you to think of any additions you might have to this list.